TANG Yun-feng, CHAI Qin-qin, LIN Shuang-jie, HUANG Jie, LI Yu-rong, WANG Wu. Study on Detection System of Grape Seed Oil Adulteration Based on Visible/Near Infrared Spectroscopy[J]. Spectroscopy and Spectral Analysis, 2020, 40(1): 202

Search by keywords or author
- Spectroscopy and Spectral Analysis
- Vol. 40, Issue 1, 202 (2020)
Abstract
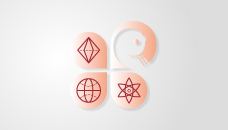
Set citation alerts for the article
Please enter your email address