Hao Peng, Wanqi Wang, Long Chen, Xianrong Peng, Jianlin Zhang, Zhiyong Xu, Yuxing Wei, Meihui Li. Few-shot object detection via online inferential calibration[J]. Opto-Electronic Engineering, 2023, 50(1): 220180

Search by keywords or author
- Opto-Electronic Engineering
- Vol. 50, Issue 1, 220180 (2023)
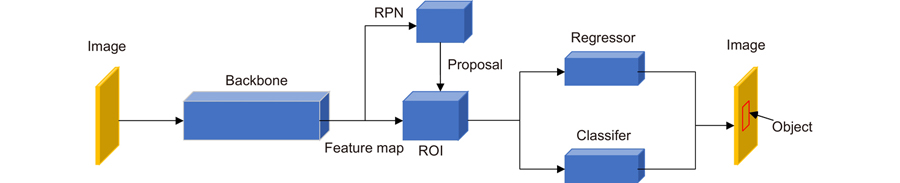
Set citation alerts for the article
Please enter your email address