SHENG Xiao-hui, LI Zi-wen, LI Zong-peng, ZHANG Fu-yan, ZHU Ting-ting, WANG Jian, YIN Jian-jun1, SONG Quan-hou. Determination of Korla Pear Hardness Based on Near-Infrared Spectroscopy[J]. Spectroscopy and Spectral Analysis, 2019, 39(9): 2818

Search by keywords or author
- Spectroscopy and Spectral Analysis
- Vol. 39, Issue 9, 2818 (2019)
Abstract
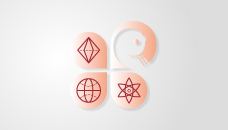
Set citation alerts for the article
Please enter your email address