
Search by keywords or author
- Spectroscopy and Spectral Analysis
- Vol. 42, Issue 9, 2836 (2022)
Abstract
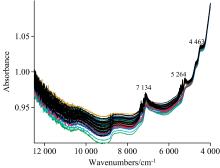
Download Citation
Set citation alerts for the article
Please enter your email address