You-rui SUN, Mei GUO, Gui-shan LIU, Nai-yun FAN, Hao-nan ZHANG, Yue LI, Fang-ning PU, Shi-hu YANG, Hao WANG. Fusion of Visible Near-Infrared (VNIR) Hyperspectral Imaging and Texture Feature for Prediction of Total Phenolics Content in Tan Mutton[J]. Spectroscopy and Spectral Analysis, 2022, 42(11): 3631

Search by keywords or author
- Spectroscopy and Spectral Analysis
- Vol. 42, Issue 11, 3631 (2022)
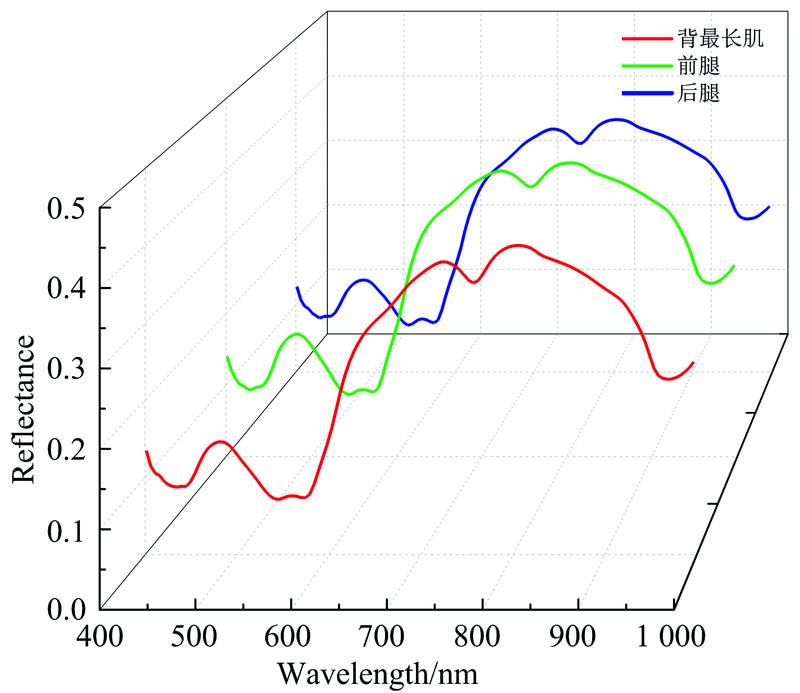
Fig. 1. Spectral curves of Tan mutton samples
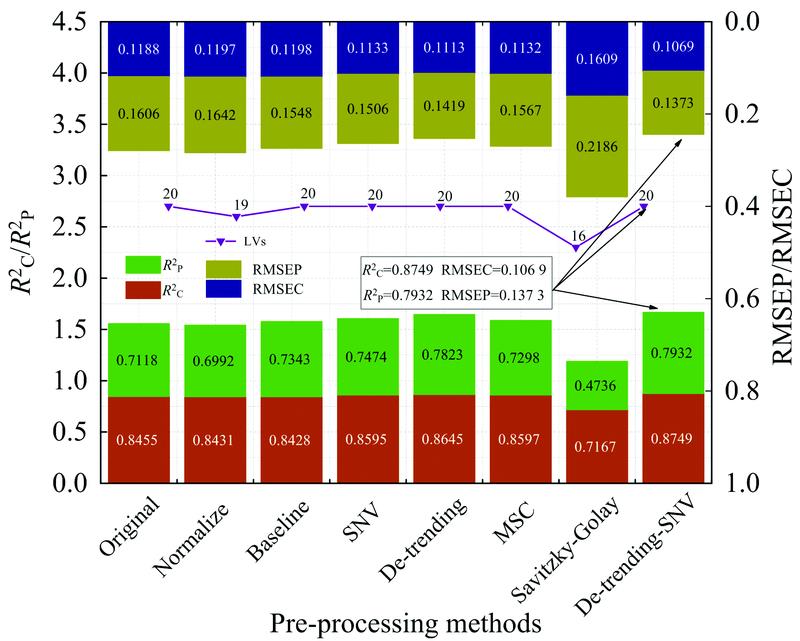
Fig. 2. Performances of full wavelength models based on different pre-processing methods
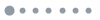
Fig. 3. Selection of the characteristic wavelengths
(a): Change curve of mean weight value and the distribution map based on BOSS algorithm;(b): Change curve of mean weight value by CARS algorithm; (c): Changes map of RMSECV by VCPA-IRIV algorithm;(d): Distribution maps based on the characteristic wavelengths extracted by VCPA-IRIV and iVISSA
(a): Change curve of mean weight value and the distribution map based on BOSS algorithm;(b): Change curve of mean weight value by CARS algorithm; (c): Changes map of RMSECV by VCPA-IRIV algorithm;(d): Distribution maps based on the characteristic wavelengths extracted by VCPA-IRIV and iVISSA
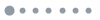
Fig. 4. The images of first three PCs of mutton samples
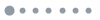
Fig. 5. Visualizaion maps of TPC content distributions
|
Table 1. Model performances based on different feature-wavelength methods
|
Table 2. Model performance based on image and spectroscopy fusion
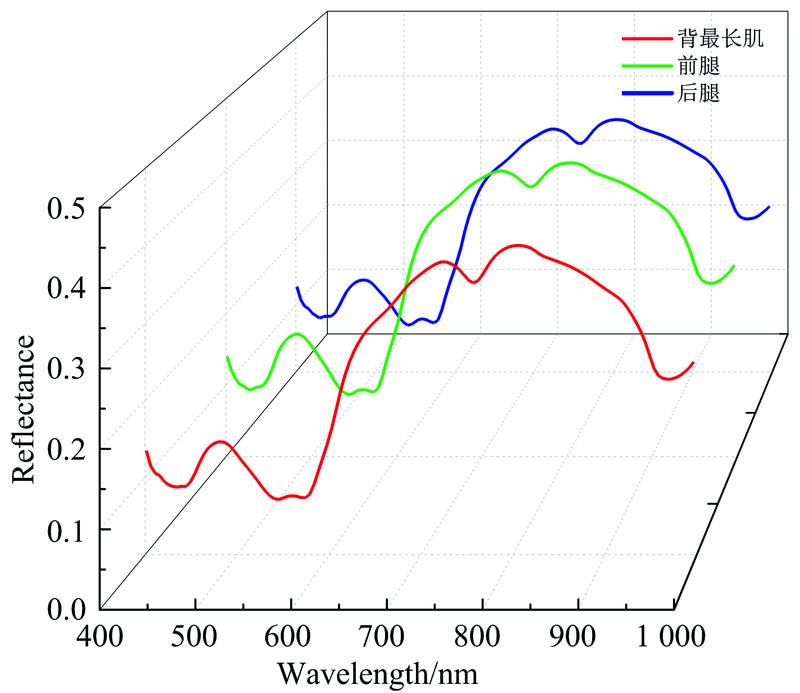
Set citation alerts for the article
Please enter your email address