You-rui SUN, Mei GUO, Gui-shan LIU, Nai-yun FAN, Hao-nan ZHANG, Yue LI, Fang-ning PU, Shi-hu YANG, Hao WANG. Fusion of Visible Near-Infrared (VNIR) Hyperspectral Imaging and Texture Feature for Prediction of Total Phenolics Content in Tan Mutton[J]. Spectroscopy and Spectral Analysis, 2022, 42(11): 3631

Search by keywords or author
- Spectroscopy and Spectral Analysis
- Vol. 42, Issue 11, 3631 (2022)
Abstract
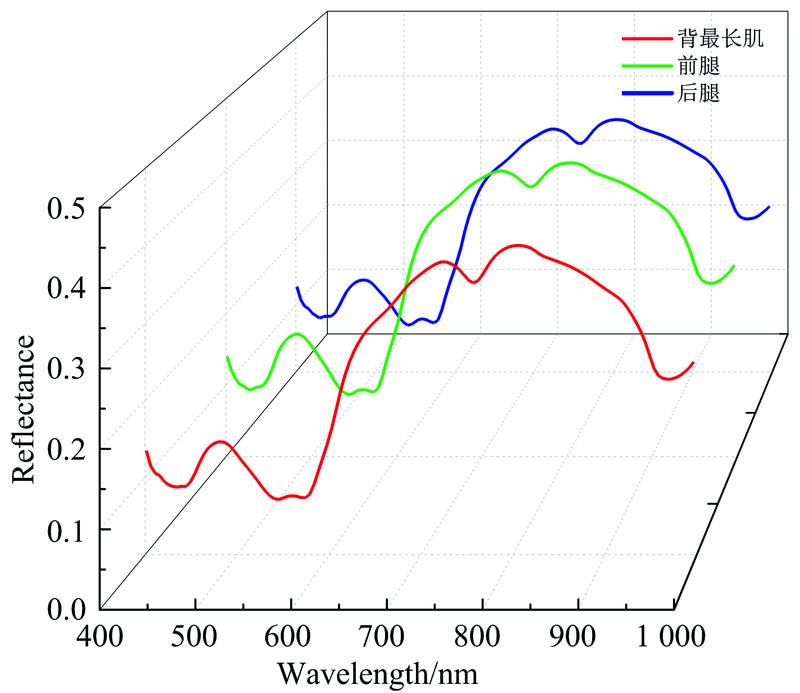
Set citation alerts for the article
Please enter your email address