Rui LI, Bo LI, Xue-wen WANG, Tao LIU, Lian-jie LI, Shu-xiang FAN. A Classification Method of Coal and Gangue Based on XGBoost and Visible-Near Infrared Spectroscopy[J]. Spectroscopy and Spectral Analysis, 2022, 42(9): 2947

Search by keywords or author
- Spectroscopy and Spectral Analysis
- Vol. 42, Issue 9, 2947 (2022)
Abstract
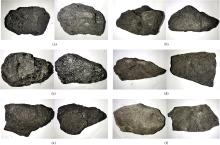
Set citation alerts for the article
Please enter your email address