YANG Sai, ZHU Qi-bing, HUANG Min. Application of Joint Skewness Algorithm to Select Optimal Wavelengths of Hyperspectral Image for Maize Seed Classification[J]. Spectroscopy and Spectral Analysis, 2017, 37(3): 990

Search by keywords or author
- Spectroscopy and Spectral Analysis
- Vol. 37, Issue 3, 990 (2017)
Abstract
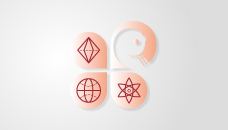
Set citation alerts for the article
Please enter your email address