Ze-Hao Wang, Long-Kun Shan, Tong-Tian Weng, Tian-Long Chen, Xiang-Dong Chen, Zhang-Yang Wang, Guang-Can Guo, Fang-Wen Sun, "Learning the imaging mechanism directly from optical microscopy observations," Photonics Res. 12, 7 (2024)

Search by keywords or author
- Photonics Research
- Vol. 12, Issue 1, 7 (2024)
Abstract
(A1) |
View in Article
(B1) |
View in Article
(B2) |
View in Article
(B3) |
View in Article
(D1) |
View in Article
(D2) |
View in Article
(D3) |
View in Article
(D4) |
View in Article
(F1) |
View in Article
(F2) |
View in Article
(F3) |
View in Article
(F4) |
View in Article
(F5) |
View in Article
(F6) |
View in Article
(I1) |
View in Article
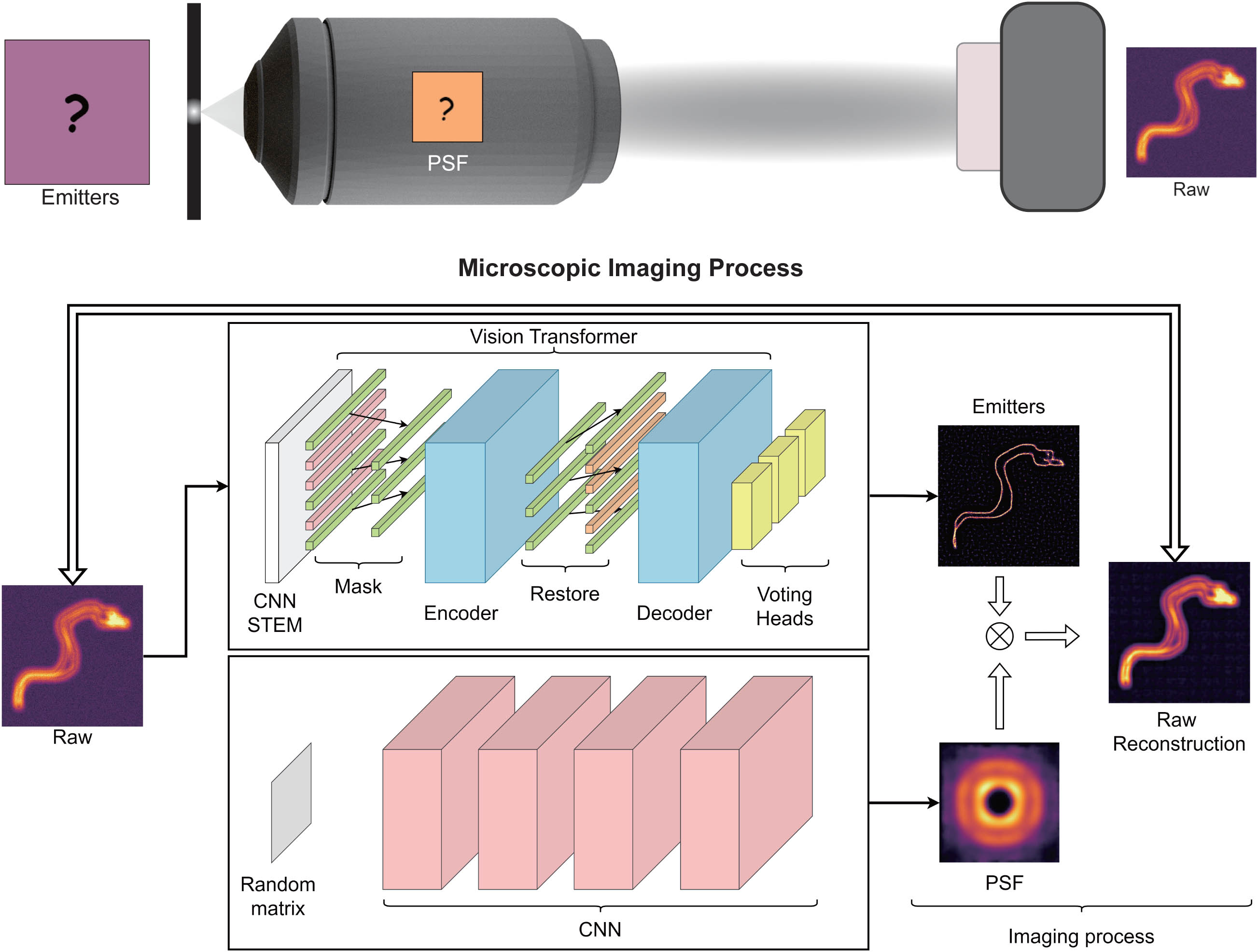
Set citation alerts for the article
Please enter your email address