LIU Tian-ling, SU Qi-ya, SUN Qun, YANG Li-ming. Recognition of Corn Seeds Based on Pattern Recognition and Near Infrared Spectroscopy Technology[J]. Spectroscopy and Spectral Analysis, 2012, 32(6): 1550

Search by keywords or author
- Spectroscopy and Spectral Analysis
- Vol. 32, Issue 6, 1550 (2012)
Abstract
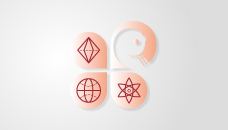
Set citation alerts for the article
Please enter your email address