[in Chinese], [in Chinese], [in Chinese], [in Chinese], [in Chinese], [in Chinese], [in Chinese]. ICA-Unet: An improved U-net network for brown adipose tissue segmentation[J]. Journal of Innovative Optical Health Sciences, 2022, 15(3): 2250018

Search by keywords or author
- Journal of Innovative Optical Health Sciences
- Vol. 15, Issue 3, 2250018 (2022)
Abstract
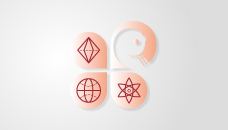
Set citation alerts for the article
Please enter your email address