ZHU Shi-chao, YOU Jian, JIN Gang, LEI Yu, GUO Xue-mei. Discrimination of Different Types of PLA with Near Infrared Spectroscopy[J]. Spectroscopy and Spectral Analysis, 2018, 38(10): 3053

Search by keywords or author
- Spectroscopy and Spectral Analysis
- Vol. 38, Issue 10, 3053 (2018)
Abstract
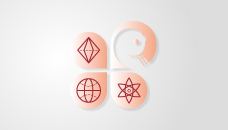
Set citation alerts for the article
Please enter your email address