CHEN Xiu-qing, YANG Qi, HAN Jing-ye, LIN Lin, SHI Liang-sheng. Estimation of Winter Wheat Leaf Water Content Based on Leaf and Canopy Hyperspectral Data[J]. Spectroscopy and Spectral Analysis, 2020, 40(3): 891

Search by keywords or author
- Spectroscopy and Spectral Analysis
- Vol. 40, Issue 3, 891 (2020)
Abstract
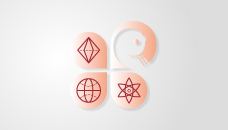
Set citation alerts for the article
Please enter your email address