Gou Yutao, Ma Liang, Song Yixuan, Jin Lei, Lei Tao. Multi-task learning for thermal pedestrian detection[J]. Opto-Electronic Engineering, 2021, 48(12): 210358

Search by keywords or author
- Opto-Electronic Engineering
- Vol. 48, Issue 12, 210358 (2021)
Abstract
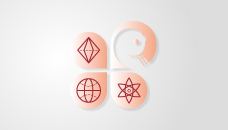
Set citation alerts for the article
Please enter your email address