Shuai HAO, Shan GAO, Xu MA, Beiyi AN, Tian HE, Hu WEN, Feng WANG. Infrared Pedestrian Detection Based on Cross-scale Feature Aggregation and Hierarchical Attention Mapping[J]. Acta Photonica Sinica, 2022, 51(6): 0610006

Search by keywords or author
- Acta Photonica Sinica
- Vol. 51, Issue 6, 0610006 (2022)
Abstract
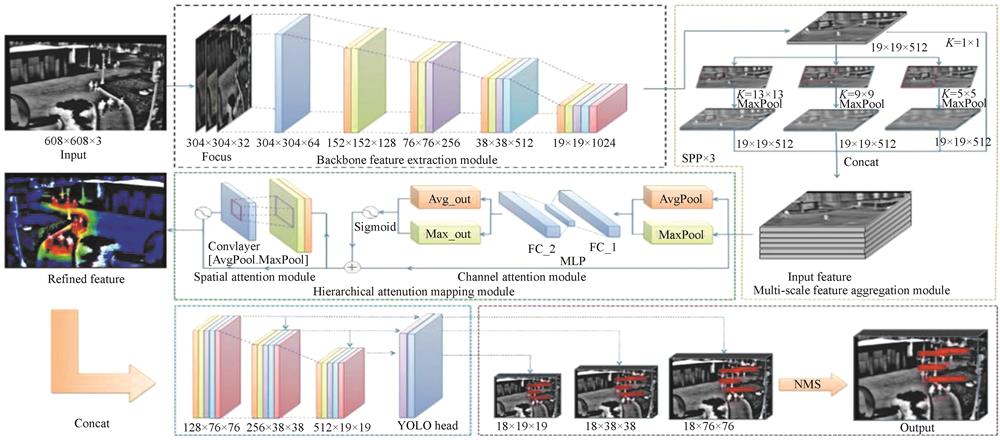
Set citation alerts for the article
Please enter your email address