Honghao Zhou, Weining Yi, Lili Du, Yanli Qiao. Convolutional Neural Network-Based Dimensionality Reduction Method for Image Feature Descriptors Extracted Using Scale-Invariant Feature Transform[J]. Laser & Optoelectronics Progress, 2019, 56(14): 141008

Search by keywords or author
- Laser & Optoelectronics Progress
- Vol. 56, Issue 14, 141008 (2019)
Abstract
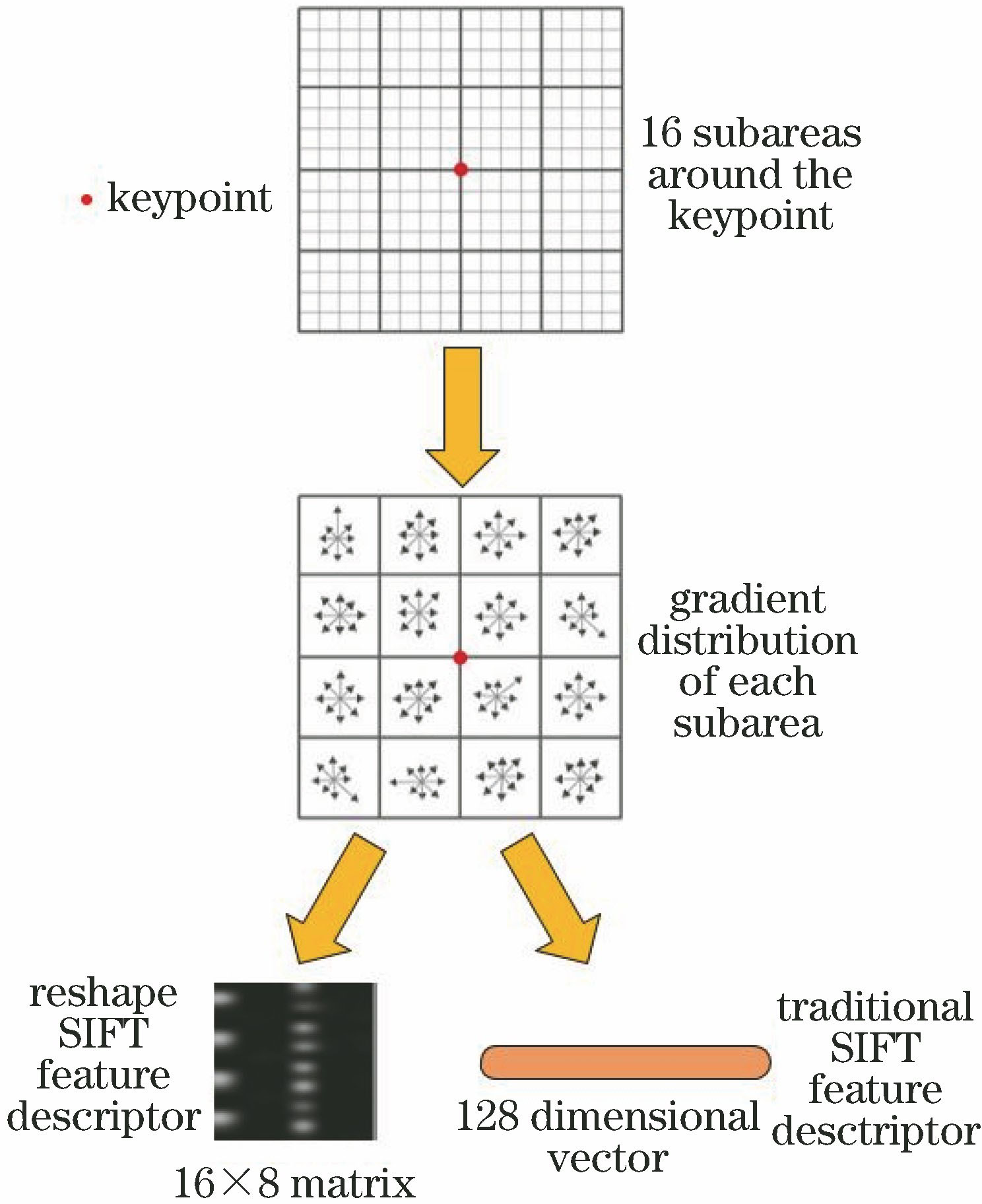
Set citation alerts for the article
Please enter your email address