Qing-lin TIAN, Bang-jie GUO, Fa-wang YE, Yao LI, Peng-fei LIU, Xue-jiao CHEN. Mineral Spectra Classification Based on One-Dimensional Dilated Convolutional Neural Network[J]. Spectroscopy and Spectral Analysis, 2022, 42(3): 873

Search by keywords or author
- Spectroscopy and Spectral Analysis
- Vol. 42, Issue 3, 873 (2022)
Abstract
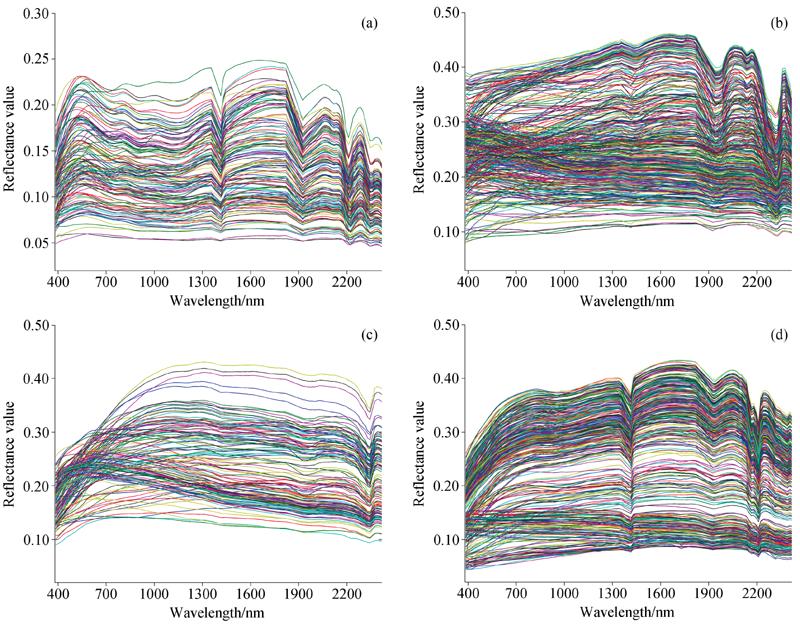
Set citation alerts for the article
Please enter your email address