Daoquan WEI, Huiqin WANG, Ke WANG, Zhan WANG, Gang ZHEN. Pigment Classification Method of Mural Sparse Multi-spectral Image Based on Space Spectrum Joint Feature[J]. Acta Photonica Sinica, 2022, 51(4): 0430002

Search by keywords or author
- Acta Photonica Sinica
- Vol. 51, Issue 4, 0430002 (2022)
Abstract
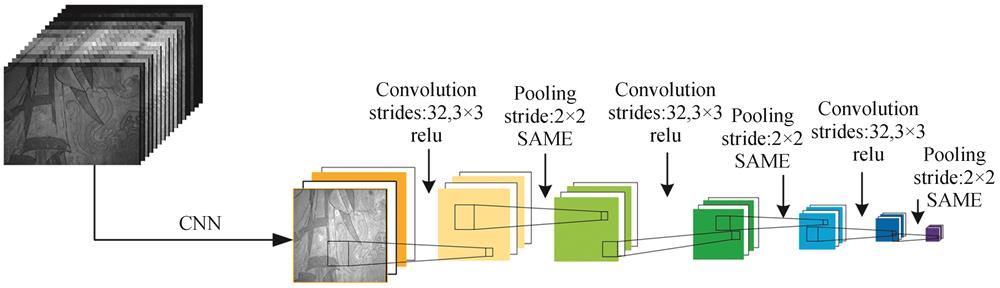
Set citation alerts for the article
Please enter your email address