ZHOU Meng-ran, LI Da-tong, HU Feng, LAI Wen-hao, WANG Ya, ZHU Song. Research of the AdaBoost Arithmetic in Recognition and Classifying of Mine Water Inrush Sources Fluorescence Spectrum[J]. Spectroscopy and Spectral Analysis, 2019, 39(2): 485

Search by keywords or author
- Spectroscopy and Spectral Analysis
- Vol. 39, Issue 2, 485 (2019)
Abstract
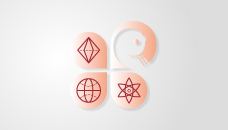
Set citation alerts for the article
Please enter your email address