LIU Zhen-wen, XU Ling-jie, CHEN Xiao-jing. Near Infrared Spectroscopy Transfer Based on Deep Autoencoder[J]. Spectroscopy and Spectral Analysis, 2020, 40(7): 2313

Search by keywords or author
- Spectroscopy and Spectral Analysis
- Vol. 40, Issue 7, 2313 (2020)
Abstract
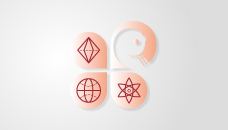
Set citation alerts for the article
Please enter your email address