HUANG Shuang-yan, YANG Liao, CHEN Xi, YAO Yuan. Study of Typical Arid Crops Classification Based on Machine Learning[J]. Spectroscopy and Spectral Analysis, 2018, 38(10): 3169

Search by keywords or author
- Spectroscopy and Spectral Analysis
- Vol. 38, Issue 10, 3169 (2018)
Abstract
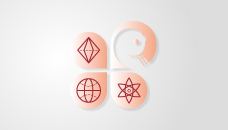
Set citation alerts for the article
Please enter your email address