[11] LI W, DU Q. Unsupervised nearest regularized subspace f anomaly detection in hyperspectral imagery[C]. 2013 IEEE International Geoscience Remote Sensing SymposiumIGARSS, Melbourne, Australia, 2013: 10551058.https:ieeexple.ieee.gdocument6721345tp=&arnumber=6721345

Search by keywords or author
- Acta Photonica Sinica
- Vol. 49, Issue 6, 0630004 (2020)
References
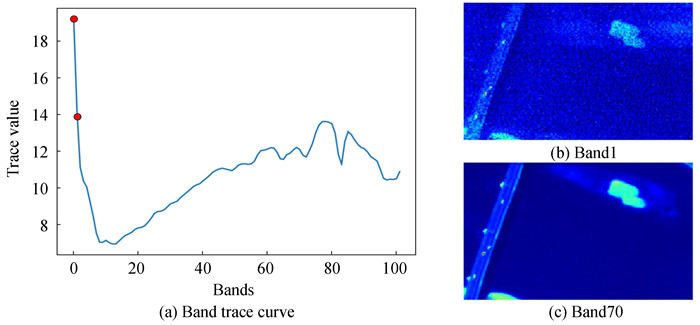
Zhi-wei WANG, Kun TAN, Xue WANG, Jian-wei DING, Yu CHEN. Unsupervised Nearest Regularized Subspace Based on Spectral Space Reconstruction for Hyperspectral Anomaly Detection[J]. Acta Photonica Sinica, 2020, 49(6): 0630004
Download Citation
Set citation alerts for the article
Please enter your email address