Zhi-wei WANG, Kun TAN, Xue WANG, Jian-wei DING, Yu CHEN. Unsupervised Nearest Regularized Subspace Based on Spectral Space Reconstruction for Hyperspectral Anomaly Detection[J]. Acta Photonica Sinica, 2020, 49(6): 0630004

Search by keywords or author
- Acta Photonica Sinica
- Vol. 49, Issue 6, 0630004 (2020)
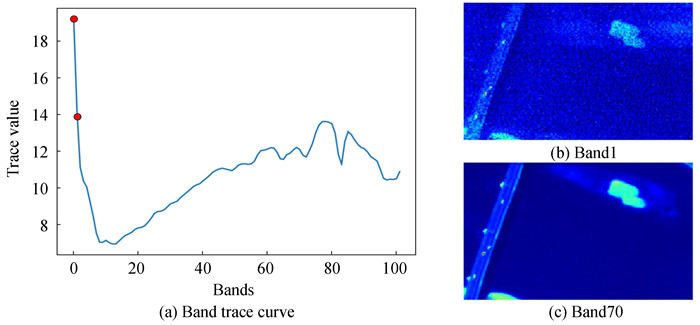
Fig. 1. Band trace curve and trace value matrix at different bands
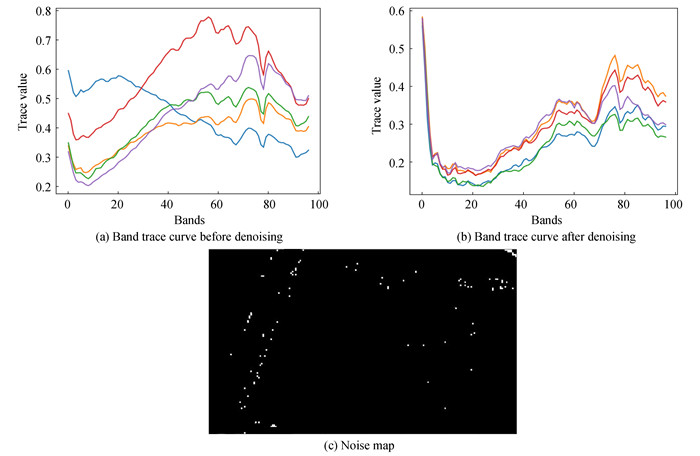
Fig. 2. Comparison of band trace curve before and after denoising and noise map
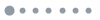
Fig. 3. The model of dual window
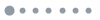
Fig. 4. Framework of the proposed method
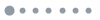
Fig. 5. AVIRIS dataset
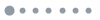
Fig. 6. ROSIS dataset
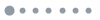
Fig. 7. Avon dataset
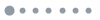
Fig. 8. GF-5 Xuzhou dataset
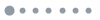
Fig. 9. Detection results of four data sets under different window sizes
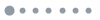
Fig. 10. Detection results of various detection algorithms in AVIRIS data
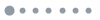
Fig. 11. Detection results of various detection algorithms in ROSIS data
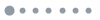
Fig. 12. Detection results of various detection algorithms in Avon data
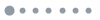
Fig. 13. Detection results of various detection algorithms in GF-5 data
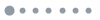
Fig. 14. ROC curves of four datasets
|
Table 1. Detection results of four datasets at different σd parameters
|
Table 2. Detection results of four datasets at different λ parameters
|
Table 3. Comparison of AUC and execution times of the different methods for four datasets
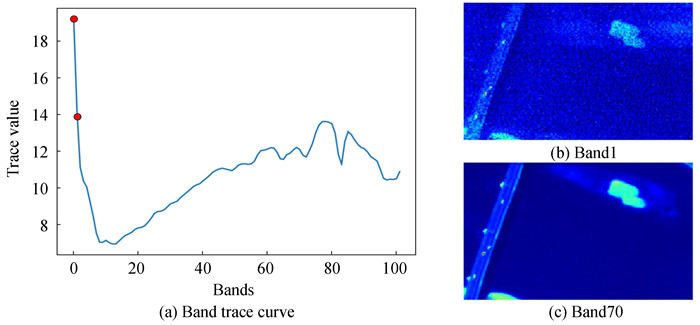
Set citation alerts for the article
Please enter your email address