LIN Ping, ZHANG Hua-zhe, HE Jian-qiang, ZOU Zhi-yong, CHEN Yong-ming. Research on Chalky Rice Detection Based on Visible Spectrogram and Deep Neural Network Technology[J]. Spectroscopy and Spectral Analysis, 2020, 40(1): 233

Search by keywords or author
- Spectroscopy and Spectral Analysis
- Vol. 40, Issue 1, 233 (2020)
Abstract
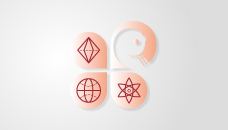
Set citation alerts for the article
Please enter your email address