Ze-huan Zheng, Sheng-ke Zhu, Ying Chen, Huanyang Chen, Jin-hui Chen. Towards integrated mode-division demultiplexing spectrometer by deep learning[J]. Opto-Electronic Science, 2022, 1(11): 220012

Search by keywords or author
- Opto-Electronic Science
- Vol. 1, Issue 11, 220012 (2022)
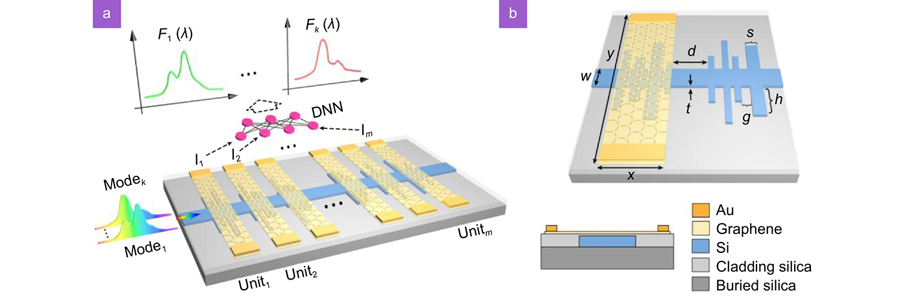
Fig. 1. (a ) Illustration of the proposed mode-division demultiplexing (MDD) spectrometer. The multimode spectral signal (mode1–modek) dispersed in the branched waveguide structures and detected by graphene-photodetector units. The generated photocurrents I1–Im at unit1–unitm from the input multimode spectral signals are fed into a deep neural network (DNN) to extract the desired spectral profiles F1 (λ)–Fk(λ). (b ) Structural parameters of a designed branched waveguide and graphene photodetector. Inset: the cross-section view of a silicon waveguide and graphene photodetector.

Fig. 2. Simulated characterizations of the proposed device for the single-mode (TE1–TE4) spectrometer by Tikhonov regularization optimization. (a ) Normalized photoresponses of two typical spectral detecting units. (b ) Correlation functions of the spectral responses for different TE modes. (c, d ) Recovered spectral profiles of dual peaks separated by 7 nm (c) and the same random spectra (d) for TE1–TE4.
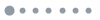
Fig. 3. Principle of the single-shot multimode reconstruction (SMR) algorithm. The unknown multimode spectra are imported to the proposed MDD spectrometer at the same time. The response photocurrents I1-Imare captured at unit1-unitm, and then are fed into the trained SMR-DNN as a pixel image to implement simultaneous reconstruction of different mode spectra in single shot.
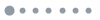
Fig. 4. Single-shot simultaneous multimode reconstructions from the trained SMR-DNN for TE1–TE4. (a ) Dual spectral peaks resolution with light wavelength separated by 15 nm, (b ) single peaks with FWHM-15 nm uniformly distributed in the bandwidth of 1500-1600 nm. (c –f ) The construction of multimode spectra in different modes: (c) TE1, (d) TE2, (e) TE3, and (f) TE4.
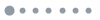
Fig. 5. Principle and performance of the designed multi-shot resolution-enhanced (MRE) architecture. (a ) Principle of the MRE algorithm. The various single-mode photocurrent (T1–Tk) images are generated by the spectral responses of photodetecting arrays and are fed into MRE-DNN to obtain resolution-improved results. (b –d ) Recovered results of dual spectral peaks with light wavelength separated by 3 nm (b), single peaks with FWHM-3 nm uniformly distributed in the bandwidth (c ), and the constructed random spectra (d).
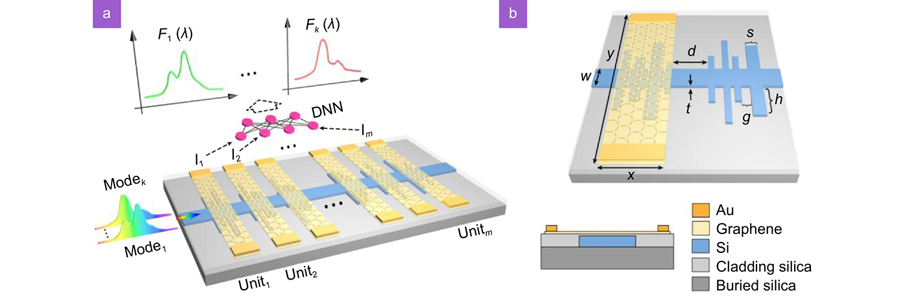
Set citation alerts for the article
Please enter your email address