Kewang Deng, Huijie Zhao, Na Li, Hui Cai. Improved data-driven compressing method for hyperspectral mineral identification models[J]. Infrared and Laser Engineering, 2022, 51(3): 20210252

Search by keywords or author
- Infrared and Laser Engineering
- Vol. 51, Issue 3, 20210252 (2022)
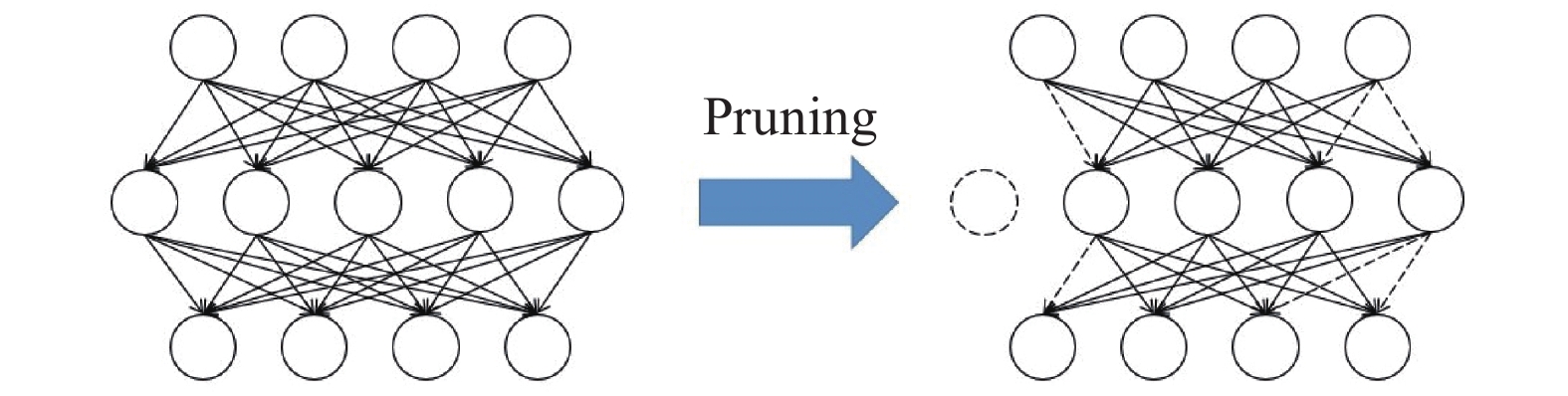
Fig. 1. Schematic of network pruning
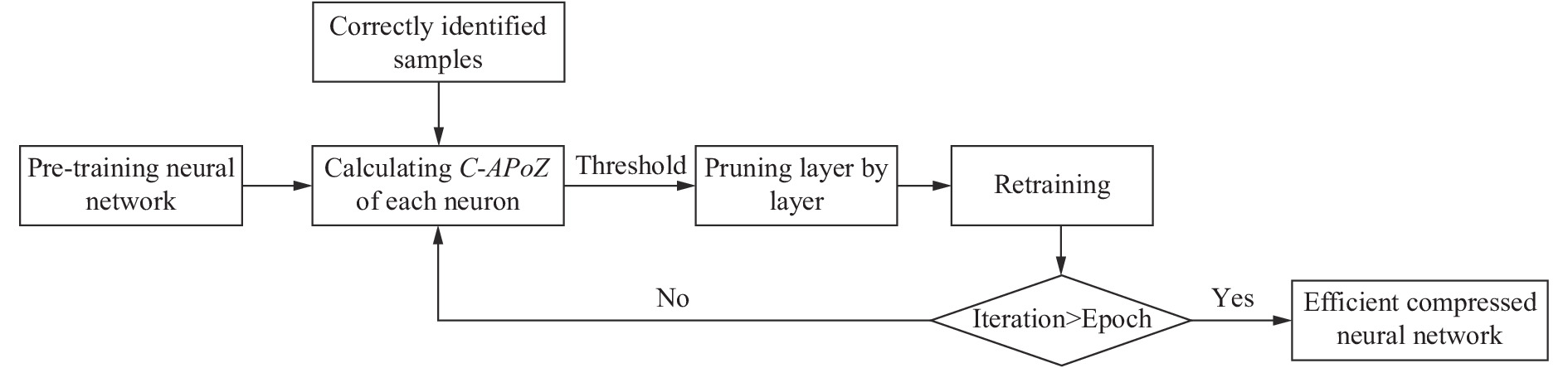
Fig. 2. Flow chart of improved sample-driven compression method for hyperspectral mineral identification model
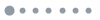
Fig. 3. Hyperspectral datasets of the Cuprite mine in Nevada
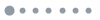
Fig. 4. Importance diagram of neurons in hidden layer
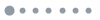
Fig. 5. Output results of the pruned neurons
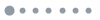
Fig. 6. Parameters of the compressed identification models obtained in the iterative pruning process
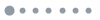
Fig. 7. Identification results of the Nevada mining area
|
Table 1. Information of identified minerals
|
Table 2. Model identification accuracy corresponding to the different number of hidden units
|
Table 3. Result chart of different pruning methods
|
Table 4. Iterative pruning results based on different importance criteria
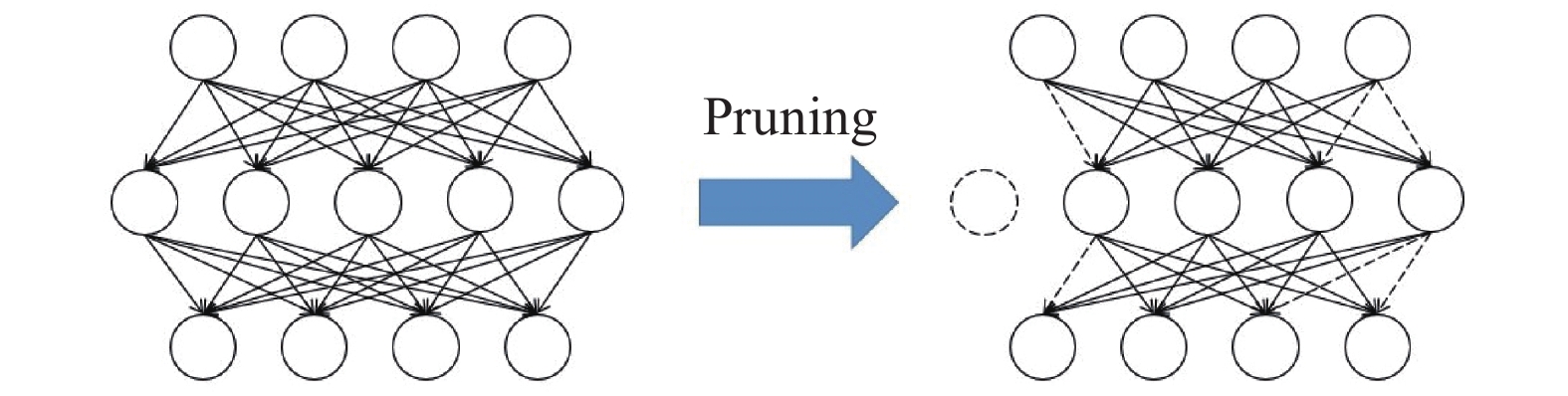
Set citation alerts for the article
Please enter your email address