LI Wei, LI Jin-long, LI Wei-jun, LIU Li-wei, LI Hao-guang, CHEN Chen, CHEN Shao-jiang. Near Infrared Spectroscopy Analysis Based Machine Learning to Identify Haploids in Maize[J]. Spectroscopy and Spectral Analysis, 2018, 38(9): 2763

Search by keywords or author
- Spectroscopy and Spectral Analysis
- Vol. 38, Issue 9, 2763 (2018)
Abstract
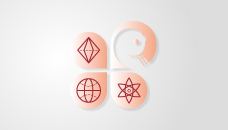
Set citation alerts for the article
Please enter your email address