Yanwei Zhang, Song Lang, Xuan Cao, Hanqing Zheng, Yan Gong. Deep neural network based on multi-level wavelet and attention for structured illumination microscopy[J]. Journal of Innovative Optical Health Sciences, 2024, 17(2): 2350015

Search by keywords or author
- Journal of Innovative Optical Health Sciences
- Vol. 17, Issue 2, 2350015 (2024)
Note: This section is automatically generated by AI . The website and platform operators shall not be liable for any commercial or legal consequences arising from your use of AI generated content on this website. Please be aware of this.
Abstract
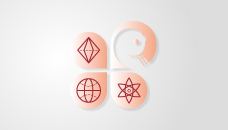
Set citation alerts for the article
Please enter your email address