ZHANG Ling-xian, TIAN Xiao, LI Yun-xia, CHEN Yun-qiang, CHEN Ying-yi, MA Jun-cheng. Estimation of Disease Severity for Downy Mildew of Greenhouse Cucumber Based on Visible Spectral and Machine Learning[J]. Spectroscopy and Spectral Analysis, 2020, 40(1): 227

Search by keywords or author
- Spectroscopy and Spectral Analysis
- Vol. 40, Issue 1, 227 (2020)
Abstract
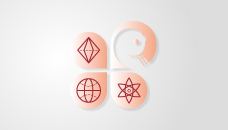
Set citation alerts for the article
Please enter your email address