Abstract
The Abbe diffraction limit, which relates the maximum optical resolution to the numerical aperture of the lenses involved and the optical wavelength, is generally considered a practical limit that cannot be overcome with conventional imaging systems. However, it does not represent a fundamental limit to optical resolution, as demonstrated by several new imaging techniques that prove the possibility of finding the subwavelength information from the far field of an optical image. These include super-resolution fluorescence microscopy, imaging systems that use new data processing algorithms to obtain dramatically improved resolution, and the use of super-oscillating metamaterial lenses. This raises the key question of whether there is in fact a fundamental limit to the optical resolution, as opposed to practical limitations due to noise and imperfections, and if so then what it is. We derive the fundamental limit to the resolution of optical imaging and demonstrate that while a limit to the resolution of a fundamental nature does exist, contrary to the conventional wisdom it is neither exactly equal to nor necessarily close to Abbe’s estimate. Furthermore, our approach to imaging resolution, which combines the tools from the physics of wave phenomena and the methods of information theory, is general and can be extended beyond optical microscopy, e.g., to geophysical and ultrasound imaging.1 Introduction
High-resolution optical imaging holds the key to the understanding of fundamental microscopic processes both in nature and in artificial systems—from the charge carrier dynamics in electronic nanocircuits1 to the biological activity in cellular structures.2 However, optical diffraction prevents the “squeezing” of light into dimensions much smaller than its wavelength,3 leading to the celebrated Abbe diffraction limit.4–7 This does not allow a straightforward extension of the conventional optical microscopy to the direct imaging of such subwavelength structures as cell membranes, individual viruses, or large protein molecules. As a result, recent decades have seen an increasing interest in developing “super-resolution” optical methods that allow to overcome this diffraction barrier—i.e., near-field optical microscopy,8 structured illumination imaging,9 metamaterials-based super-resolution,10 two-photon luminescence and stimulated emission-depletion microscopy,11 stochastic optical reconstruction imaging,12 and photoactivated localization microscopy.13
In particular, there is an increasing demand for the approach to optical imaging that is inherently label-free and does not rely on fluorescence, operates on the sample that is in the far field of all elements of the imaging system, and offers resolution comparable to that of fluorescent microscopy. Although seemingly a tall order, this task has recently found two possible solutions that approach the problem from the “hardware” and “algorithmic” sides, respectively. The former approach relies on the phenomenon of “super-oscillations”—where the band-limited function can and—when properly designed—does oscillate faster than its fastest Fourier component. The super-oscillatory lenses that implement this behavior have been designed and fabricated,14,15 and optical resolution exceeding the conventional Abbe limit has been demonstrated in experiment.14 The second approach relies on methods of processing the “diffraction-limited” data, taking full advantage of the fact that actual targets (and especially biological samples) are often inherently sparse.3 The resulting resolution improvement beyond the Abbe limit, due to this improved data processing, has been demonstrated both in numerical simulations and in experiment.16–18
Far-field optical resolution beyond the Abbe limit in a scattering rather than fluorescence-based approach, observed in Refs. 14–19, clearly demonstrates that Abbe’s bound of half-wavelength (and its quarter-wavelength counterpart for structured illumination) is not a fundamental limit for optical imaging. This raises the key question of whether there is in fact a fundamental bound to the optical resolution—as opposed to practical limitations due to detector noise, imaging system imperfections, data processing time limits in the case when image reconstruction corresponds to an NP-complete problem, etc. Furthermore, the knowledge of the corresponding fundamental limit, if such exists, and the physical mechanism behind it would help find the way to the system that offers the optimal performance—just as deeper understanding of thermodynamics and Carnot’s limit helped the design of practical heat engines.
Sign up for Advanced Photonics TOC. Get the latest issue of Advanced Photonics delivered right to you!Sign up now
In this work, we show that there is in fact a fundamental limit on the resolution of far-field optical imaging, which is however much less stringent than Abbe’s criterion. The presence of any finite amount of noise in the system, regardless of how small its intensity, leads to a fundamental limit on the optical resolution, which can be expressed in the form of an effective uncertainty relation. This limit has an essential information-theoretical nature and can be connected to the Shannon’s theory of information transmission in linear systems.20
2 Definition of the Resolution Limit
We define the diffraction limit as the shortest spatial scale of the object whose geometry can still be reconstructed, error-free, from the far-field optical measurements in the presence of noise. (Although the concept of error-free information recovery in the presence of noise may sound surprising, it lies in the heart of modern computer networks where terabytes of data are transferred error-free over noisy transmission lines.) Without loss of generality, one can then assume that the object is composed of an arbitrary number of point scatterers of arbitrary amplitudes located at the nodes of the grid with the period , as any additional structure in the sources (or scatterers) or variations in position will add to the information that needs to be recovered from far-field measurement for the successful reconstruction of the geometry of the object. (For a given illumination field, each point scatterer can be treated as an effective point source.)
Furthermore, the essential “lower bound” nature of further allows to reduce the problem to that of an effectively one-dimensional target (formed by line, rather than point, sources)—since, as was already known to André21 and Rayleigh,22 line sources are more easily resolvable than point sources.
To calculate the fundamental resolution limit, it is therefore sufficient to consider the model system of an array of line “sources” of arbitrary (including zero) amplitudes, located at the node points of the grid with the period [see Fig. 1(a)]. Note that in terms of the information that is detected in the far field and the information that is necessary and sufficient for the target reconstruction, this problem is identical to that of a step mask where thickness and/or permittivity changes at the nodes of the same grid by the amounts proportional to the amplitudes of the corresponding line sources (as the point source distribution corresponds to the spatial derivative of the mask “profile”) [see Fig. 1(b)].
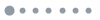
Figure 1.The schematic representation of the imaging set-up, for the object formed by (a) an array of small particles/lines and (b) a (binary) mask. labels the position of a (coherent) detector, is the size of the object (and equivalently the imaging aperture), and is the distance from the object to the detector; in the far field .
Note that the reduction of the original problem to that of an effectively one-dimensional profile is not a simplification for the sake of convenience or reduction of the mathematical complexity. It is exactly this “digitized” one-dimensional profile that corresponds to the smallest “resolvable” spatial scale among all objects with a low bound on their spatial variations and therefore defines the fundamental resolution limit. Furthermore, in many cases, the actual object is formed by two (or more) materials that form sharp interfaces. In this case, the step mask that is equivalent to our point source model offers an adequate representation of the actual target.
However, even within the original framework of “resolving” two point sources,22 the result clearly depends on the difference of their amplitudes—with increasing disparity between the two leading to progressively worse “resolution.” The “ultimate” resolution limit , therefore, corresponds to the case of identical point sources (or subwavelength scatterers), which are present only in an (unknown) fraction of the grid nodes. Note that such a digital mask corresponds to the common case of a pattern formed by a single material (e.g., the surrounding air) [see Fig. 1(b)].
When the distance to the detector is much larger than the aperture , (see Fig. 1), for the far-field signal detected in the given polarization and in the direction defined by the wavevector (see Fig. 1 and Sec. 7): where is the incident field “illuminating” the target, is the (integer) index that labels the (point) scatterers with the corresponding polarizabilities , , is the wavevector with the magnitude , is the light frequency, and is the speed of light (in the medium surrounding the target). Here corresponds to the effective noise, which includes the contributions from all origins (detector dark currents, illumination field fluctuations, etc.). Using data for imaging with different electromagnetic field polarizations, the effective noise can be correspondingly reduced.
Equivalently, for the case of the object in the form of a (dielectric) mask [see Fig. 1(b)], we obtain where is the difference between the dielectric permittivities of the object and the background.
Note that Eqs. (1) and (2) are linear in and (see Sec. 7), which physically correspond to the limit when multiple scattering is weak. Although this is generally the case in optical imaging of low-contrast media, secondary waves due to multiple light scattering can be intentionally induced by an a priori known high-contrast grating placed in the near field of the object.23–25 Such grating-assisted microscopy offers a substantial improvement of imaging resolution well beyond what is expected for conventional far-field imaging.23–25
The model of Eq. (1) or its equivalent Eq. (2) assumes coherent detection of the electromagnetic field in the far zone. This is essential for the definition of the fundamental resolution limit, as the phase information is in fact available in the far field and can be measured even with an intensity only sensitive detector using optical heterodyne approach26 so that any failure to obtain the corresponding information in a given experimental setup cannot be attributed to the fundamental resolution limit of optical imaging.
Finally, for the calculation of the fundamental resolution limit , we must assume the large aperture limit . Although the case of a small aperture can be easily implemented in the actual experimental setup (albeit at the cost of dramatic reduction in the field of view), the aperture in a close proximity to the object represents an example of a near-field probe, and this setup cannot be treated as a true far-field imaging.
3 Information-Theoretical Framework
To derive the fundamental limit on the resolution of optical imaging, we calculate the total amount of information about the object that can be recovered in the far field. As Eq. (1) can be interpreted as the input ()–output relation of a linear information channel, the amount of the actual information carried from the object to the far-field detector, can be calculated using the standard methods of the information theory.20 The resolution limit then follows from the requirement of the recovered information being sufficient to reconstruct the target:
When the object is composed of different materials (or is formed by an array of point sources with different levels of amplitude), additional information is needed for its reconstruction, which leads to a more stringent bound on the spatial resolution:
The actual transmitted information can be obtained from the mutual information functional20
Here the entropy is the measure of the information received at the detector array: where is the distribution function of the output signal , and the functional integral is defined in the standard way [see Eq. (22) in Sec. 9].
However, as the system is noisy, for any output signal, there is some uncertainty of what was the originating field scattered by the mask. The conditional entropy at the detector array for a given represents this uncertainty:
Substituting the resulting analytical expressions for and (see Sec. 9) into the mutual information in Eq. (5), for the resolution limit in the case of uniform illumination (see Sec. 10 for resolution limit in the regime of structured illumination), we obtain which in the appropriate limits is consistent with the results of the earlier information-theoretical studies of Refs. 27–30. Here SNR is the effective signal-to-noise ratio measured at the detector array: and represents the relative contribution of the absorption in the target; for a transparent object [], we have . The correction accounts for the finite size of the imaging aperture and can be neglected for .
4 Discussion
Although Eq. (8) allows for an unlimited resolution in a noise-free environment, even a relatively low noise dramatically alters this picture. With the weak logarithmic dependence of the resolution limit on the SNR, to reduce the resolution limit by a factor of ten, the SNR needs to be increased by nearly five orders of magnitude.
At the same time, the spatial resolution limit depends on the effective “uncertainty” in the range of permittivity variations in the object that is being imaged—the simpler is the structure of the target, the easier is the task of finding its geometry. The ultimate value is then achieved in the case of a binary mask (i.e., the object that is formed by only two materials) and represents the fundamental bound to the resolution. In the case of a higher complexity in the composition of the target, the actual resolution limit is well above . When the number of materials (with their corresponding permittivities) that the object is composed of, , is known a priori, the corresponding resolution limit is defined by Eq. (4). However, when no a priori information whatsoever is available, the limit to the resolution can be expressed as the effective uncertainty relation, which offers the lower bound on the product of the scaled spatial resolution and the amplitude resolution . In the case when the object is composed of transparent materials , we obtain where , the scaled spatial resolution is defined as the ratio of to the Abbe limit, and the scaled amplitude resolution corresponds to the uncertainty in the permittivity that is normalized to the difference between the smallest () and largest () permittivities in the object, . For a binary mask, so that the scaled amplitude resolution and , which reduces the uncertainty relation, Eq. (11), to the fundamental limit of Eq. (8).
For imaging with no a priori information, with the optimal data reconstruction algorithm, Eq. (11) represents a trade-off between the uncertainties in position and the amplitude of the recovered image. Note that, as follows from Eq. (11), spatial resolution at the Abbe limit corresponds to the relative amplitude uncertainty of at least .
In the case of imaging a binary mask or a pattern of identical subwavelength particles, the actual resolution can reach the value of , which for a high SNR can be substantially below the Abbe limit. For example, in the structured illumination setup with , we find . Although reaching all the way to this limit with the data obtained in the standard imaging setup may be highly nontrivial, a straightforward algorithm described below that implements the amplitude constraint, offers spatial resolution well below the Abbe limit (see Fig. 2).
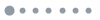
Figure 2.Super-resolution object reconstruction for a binary mask, from its coherently detected diffraction pattern in the far field. The inset shows the schematics of the object profile. The main panel plots the error probability in the recovered profile, as a function of the effective SNR. The data shown were obtained for 10,000 different realizations. The boundary separating the light-red and light-green background corresponds to the value of the SNR corresponding to sufficient to resolve the spacing (see the inset). The red arrow indicates the minimum value of SNR when the numerical reconstruction produces no errors.
In the algorithm whose performance is shown in Fig. 2, the subwavelength binary mask (see the inset in Fig. 2) is recovered from its (band-limited) Fourier spectrum measured in the far field, together with the constraint that limits its profile to only two values. Although a finite amount of noise in the far-field measurements inevitably leads to errors, with the increase of the effective SNR, the corresponding error probability rapidly goes to zero. In particular, for the resolution of in the example of Fig. 2, for the SNR beyond the value indicated by the red arrow, the numerical calculation with an ensemble of 10,000 different realizations showed no errors.
The light-red and light-green color backgrounds in Fig. 2 correspond to the parameter range that, respectively, violates and satisfies the fundamental resolution limit of Eq. (8). Note that the boundary separating these regimes corresponds to the SNR that is substantially less than the smallest value (shown by red arrow in Fig. 2) for the error-free performance in the data recovery—indicating that the reconstruction algorithm is far from optimal. Still, even with this performance, the example of Fig. 2 indicates that even a straightforward implementation of an a priori constraint on the object geometry (binary mask rather than an arbitrary profile) offers object reconstruction from diffraction-limited data with deep subwavelength resolution (four times below the Abbe limit in the example of Fig. 2).
Additional a priori information about the object further reduces the resolution limit of optical imaging. For different cases of a priori available information about the target, the case of sparse objects is particularly important, as this property is widespread in both natural and artificial systems.3 If the target is a priori known to be sparse, with the effective sparsity parameter (which can be defined as the fraction of empty “slots” in the grid superimposed on the target), we find
For the numerical example studied in Ref. 16, with and , the resolution limit . Accurate numerical reconstruction of the features on the scale of demonstrated in Ref. 16 is, therefore, fully consistent with the fundamental limit .
5 Imaging with a Small Aperture
The explicit expression for the resolution limit in Eq. (8) is presented in the large amplitude limit . Although this corresponds to the most common regime of actual optical microscopy, using a small aperture that is comparable to the free space wavelength can offer its own advantages. The resulting effect on the resolution limit is accounted for by the (positive definite) term in Eq. (8), which further reduces .
Note that this was precisely the regime where super-oscillation-based imaging was demonstrated in experiment, as the use of small aperture was essential to block the (exponentially) strong power side lobes. Although the resulting improvement of the resolution is consistent with the fundamental limit established in this work, our expressions Eqs. (4), (8), and (12) do not explicitly indicate the advantage of super-oscillation approach. This should be contrasted to the case of sparsity-based imaging where its key parameter explicitly enters the resolution limit in Eq. (12).
Indeed, while the super-oscillation imaging does offer subwavelength resolution, this improvement is the general feature of all structured illumination methods optimized for small aperture (or equivalently for imaging small isolated objects) and is not limited to the super-oscillation approach. This behavior is illustrated in Fig. 3, where a subwavelength target [red pattern in the center of Fig. 3(a)] is illuminated by the Bessel beam propagating in the direction normal to the plane of the picture. The beam axis is “focused” to the center of the target [see Fig. 3(a)] so that the illuminating field within the aperture not only shows no super-oscillations but in fact does not oscillate at all—see the field profiles for different orders of the illuminating Bessel beams in Fig. 3(b). Nevertheless, the standard data recovery algorithm clearly shows deep subwavelength resolution of [see Fig. 3(c)], despite having no a priori information about the structure of the target.
![Super-resolution imaging of a subwavelength object, based on structured illumination with Bessel beams. (a) The “incident” Bessel beam of the order m=12 (shown in gray scale) focused at the center of the subwavelength object (red). (b) The Bessel beam profiles in the object plane, for different orders m=0 (red), 1 (orange), 2 (magenta), 3 (blue), 4 (cyan), and 5 (green). For a small distance from the center, the Bessel function of order m behaves as xm, so illumination with the Bessel beams of different orders effectively “projects” the target on the set {xm} for different values of m. As the latter form a complete basis set, this procedure allows high-resolution reconstruction of the original object profile, without any use of super-oscillations or subwavelength focusing. (c) The subwavelength object profile and its reconstruction with Bessel beam illumination. The object corresponds to the red line in (c). The reconstructed profiles are shown for the effective SNRs of 106 [blue line in (c)] and 104 [green line in (c)].](/Images/icon/loading.gif)
Figure 3.Super-resolution imaging of a subwavelength object, based on structured illumination with Bessel beams. (a) The “incident” Bessel beam of the order (shown in gray scale) focused at the center of the subwavelength object (red). (b) The Bessel beam profiles in the object plane, for different orders (red), 1 (orange), 2 (magenta), 3 (blue), 4 (cyan), and 5 (green). For a small distance from the center, the Bessel function of order behaves as , so illumination with the Bessel beams of different orders effectively “projects” the target on the set for different values of . As the latter form a complete basis set, this procedure allows high-resolution reconstruction of the original object profile, without any use of super-oscillations or subwavelength focusing. (c) The subwavelength object profile and its reconstruction with Bessel beam illumination. The object corresponds to the red line in (c). The reconstructed profiles are shown for the effective SNRs of [blue line in (c)] and [green line in (c)].
It should, however, be noted that the super-oscillation-based approach, when implemented to form a subwavelength focus spot that is used to scan the object,14,15 is naturally suitable for optical imaging limited to incoherent detection, which offers substantial practical advantages in the actual implementation of the system.
6 Conclusions
In conclusion, we have derived the fundamental resolution limit for far-field optical imaging and demonstrated that it is generally well below the standard half-the-wavelength estimate. Our results also apply to other methods that rely on wave propagation and scattering, e.g., geophysical and ultrasound imaging.
7 Appendix A: Imaging Model
In its most general setting, the problem of (optical) imaging is essentially the reconstruction of the object profile from scattering data. The formation of the desired image of the target can be achieved using “analog” or “digital” tools, with lenses and projection screens in the former case and computational reconstruction of the object pattern on a computer screen. If the structure of the object is represented by its dielectric permittivity profile , the scattered electric field at the given frequency is defined by the vectorial Lippmann–Schwinger equation: where is the (dyadic) Green function for the medium surrounding the object, is the difference between the permittivities of the object and of the surrounding medium, and .
Alternatively, the object may be represented as a collection of small (subwavelength) particles, with the individual (tensor) polarizabilities , leading to
Note that these two formulations are essentially equivalent, as arbitrary dielectric permittivity profile can be expressed in terms of the electromagnetic response of a large group of small particles.31
Although Eqs. (13) and (14) are linear in the electrical field, when treated as inverse problems for the reconstruction of the unknown profile and the distribution from the given illumination field and the scattering data for , they are essentially nonlinear in and .32 Physically, this nonlinearity originates from multiple scattering effects within the object,33 when the actual field acting on the given object, , in addition to the incident field , also includes the contributions from the “secondary” waves scattered by the order parts of the object. Although these multiple scattering corrections can be substantial in acoustic and microwave scattering,33 for optical imaging of low-contrast media, these are generally small.34 Note, however, that when substantially present these “secondary” waves due to multiple light scattering can have a profound effect on the imaging resolution33—as the subwavelength structure of the object now functions as a high-spatial frequency grating forming an effective structured illumination pattern.
In the language of scattering theory, the conventional optical imaging and microscopy corresponds to the limit of weakly scattering semitransparent objects, which neglects multiple scattering contributions. The resulting first-order Born approximation34 reduces the acting field in the integral of Eq. (13) and the sum of Eq. (14) to the (a priori known) illumination field , thus leading to a linear inverse problem.
The resulting expressions can be further simplified in the radiation zone, when the detectors are placed in the far-field (radiation zone) of the object, , thus reducing Eq. (14) to
When the distance to the detector is much larger than the aperture , , for the far-field signal detected in the given polarization and the wavevector (see Fig. 1), we find where , with the magnitude , and is the noise in the corresponding detector (see Fig. 1).
Similarly, if the target is represented with the 2-D permittivity mask (corresponding to the Motti projection35 of the actual 3-D permittivity of the object), we obtain
8 Appendix B: Information Entropy
The entropy offers a measure of the information received by the detector that returns the value of and is a functional of the statistical distribution of :
When represents the scattered field detected in the imaging system, it is defined by the object structure and the illumination field profile. However, even in the absence of any stray light in the system, all detectors are inherently noisy. As a result, for a given detected signal, there will always be some uncertainty. This uncertainty is represented by the conditional information entropy of the detected signal for a given object, in terms of the conditional distribution :
According to the Shannon’s fundamental result,20 the resulting information about the object is then given by the mutual information
When the imaging system measures the continuous spectrum , the relevant entropies are defined by the functional integral: where for the entropy , and for the entropy , and the functional integral is defined in the standard way: where is the normalization constant.
9 Appendix C: Mutual Information
The mutual information is defined20 as the difference between the information entropy at the “output” for the unconstraint “input,” , and the information entropy of the output for fixed input [see Eq. (16)]. For additive noise, the latter is simply equal to the noise entropy: where is the noise distribution function and reduces to for uncorrelated Gaussian noise.
The unconditional output distribution is defined by both the noise and the target profile distribution . Although the latter does not necessarily reduce to a simple functional form, every single output component corresponds to a sum of many such random variables [see Eq. (16)]. The central limit theorem then implies that the “output” statistics of is described by the correlated multivariate normal distribution. Changing the path integral variables in Eq. (23) using the orthogonal transformation that diagonalizes the corresponding covariance matrix, we obtain where ’s are the eigenvalues of the discrete prolate spheroidal Slepian matrix36, with , where for a one-dimensional target, , and for a rectangular (square) aperture, . The eigenvalue spectrum of the Slepian matrix has a characteristic step shape, showing significant eigenvalues () and remaining insignificant eigenvalues () separated by a narrow transition band.37,38 The eigenvalue sum in Eq. (25) can be, therefore, calculated analytically, which together with Eqs. (5) and (24) yields Eq. (8).
10 Appendix D: Resolution Limit for Structured Illumination
In the case of structured illumination, for the resolution limit, we obtain
References
[1] E. Sakat et al. Near-field imaging of free carriers in ZnO nanowires with a scanning probe tip made of heavily doped Germanium. Phys. Rev. Appl., 8, 054042(2017).
[2] B. Herman, K. Jacobson. Optical Microscopy for Biology(1990).
[3] J. W. Goodman. Introduction to Fourier Optics(2004).
[4] J.-L. Lagrange. Sur une Loi generale d’Optique(1803).
[5] H. von Helmholtz. On the limits of the optical capacity of the microscope. Proc. Bristol Nat. Soc., 1, 435(1874).
[6] E. K. Abbe. Beiträge zur Theorie des Mikroskops und der mikroskopischen Wahrnehmung. Arch. Mikrosk. Anat., 9, 413-468(1873).
[7] E. Abbe. A contribution to the theory of the microscope, and the nature of microscopic vision. Proc. Bristol Nat. Soc., 1, 200-261(1874).
[8] B. Hecht et al. Scanning near-field optical microscopy with aperture probes: fundamentals and applications. J. Chem. Phys., 112, 7761-7774(2000).
[9] M. G. Gustafsson. Surpassing the lateral resolution limit by a factor of two using structured illumination microscopy. J. Microsc., 198, 82-87(2000).
[10] X. Zhang, Z. Liu. Superlenses to overcome the diffraction limit. Nat. Mater., 7, 435-441(2008).
[11] F. Balzarotti et al. Nanometer resolution imaging and tracking of fluorescent molecules with minimal photon fluxes. Science, 355, 606-612(2017).
[12] M. J. Rust, M. Bates, X. Zhuang. Sub diffraction-limit imaging by stochastic optical reconstruction microscopy (STORM). Nat. Methods, 3, 793-796(2006).
[13] E. Betzig et al. Breaking the diffraction barrier: optical microscopy on a nanometric scale. Science, 251, 1468-1470(1991).
[14] E. T. F. Rogers et al. A super-oscillatory lens optical microscope for subwavelength imaging. Nat. Mater., 11, 432-435(2012).
[15] G. H. Yuan, E. T. F. Rogers, N. I. Zheludev. Achromatic super-oscillatory lenses with sub-wavelength focusing. Light Sci. Appl., 6, e17036(2017).
[16] S. Gazit et al. Super-resolution and reconstruction of sparse sub-wavelength images. Opt. Express, 17, 23920-23946(2009).
[17] A. Szameit et al. Sparsity-based single-shot subwavelength coherent diffractive imaging. Nat. Mater., 11, 455-459(2012).
[18] P. Sidorenko et al. Sparsity-based super-resolved coherent diffraction imaging of one-dimensional objects. Nat. Commun., 6, 8209(2015).
[19] F. M. Huang, N. I. Zheludev. Super-resolution without evanescent waves. Nano Lett., 9, 1249-1254(2009).
[20] C. E. Shannon. A mathematical theory of communication. Bell Syst. Tech. J., 27, 379-423(1948).
[21] M. C. André. Étude de la Diffraction dans les Intruments d’Optique; son Influence sur les Observations Astronomiques. Ann. de l’École Norm. Sup., 5, 275-354(1876).
[22] L. Rayleigh. Investigations in optics, with special reference to the spectroscope. Philos. Mag. J. Sci., 8, 261-274(1879).
[23] A. Sentenac, P. C. Chaumet, K. Belkebir. Beyond the Rayleigh criterion: grating assisted far-field optical diffraction tomography. Phys. Rev. Lett., 97, 243901(2006).
[24] S. Inampudi, N. Kuhta, V. A. Podolskiy. Interscale mixing microscopy: numerically stable imaging of wavelength-scale objects with sub-wavelength resolution and far field measurements. Opt. Express, 23, 2753-2763(2015).
[25] C. M. Roberts et al. Interscale mixing microscopy: far-field imaging beyond the diffraction limit. Optica, 3, 803-808(2016).
[26] F. Le Clerc, L. Collot, M. Gross. Numerical heterodyne holography with two-dimensional photodetector arrays. Opt. Lett., 25, 716-718(2000).
[27] G. T. di Francia. Resolving power and information. J. Opt. Soc. Am., 45, 497-501(1955).
[28] P. B. Fellgett, E. H. Linfoot. On the assessment of optical images. Philos. Trans. R. Soc. Ser. A, 247, 369-407(1955).
[29] N. J. Bershad. Resolution, optical-channel capacity and information theory. J. Opt. Soc. Am., 59, 157-163(1969).
[30] E. L. Kosarev. Shannons superresolution limit for signal recovery. Inverse Prob., 6, 55-76(1990).
[31] B. T. Draine, P. J. Flatau. Discrete dipole approximation for scattering calculations. J. Opt. Soc. Am. A, 11, 1491-1499(1994).
[32] W. C. Chew. Waves and Fields in Inhomogeneous Media(1995).
[33] T. J. Cui et al. Study of resolution and super resolution in electromagnetic imaging for half-space problems. IEEE Trans. Antennas Propag., 52, 1398-1411(2004).
[34] M. Born, E. Wolf. Principles of Optics: Electromagnetic Theory of Propagation, Interference and Diffraction of Light(1999).
[35] E. Narimanov. Hyperstructured illumination. ACS Photonics, 3, 1090-1094(2016).
[36] D. Slepian. Prolate spheroidal wave functions, Fourier analysis and uncertainty. V: the discrete case. Bell Syst. Tech. J., 57, 1371-1430(1978).
[37] H. J. Landau. On the eigenvalue behavior of certain convolution equations. Trans. Am. Math. Soc., 115, 242-256(1965).
[38] D. Slepian, E. Sonnenblick. Eigenvalues associated with prolate spheroidal wave functions of zero order. Bell Syst. Tech. J., 44, 1745-1759(1965).