Zongda Liu, Liquan Dong, Yuejin Zhao, Lingqin Kong, Ming Liu. Adaptive Model Tracking Algorithm for Fast-Moving Targets in Video[J]. Acta Optica Sinica, 2021, 41(18): 1815001

Search by keywords or author
- Acta Optica Sinica
- Vol. 41, Issue 18, 1815001 (2021)
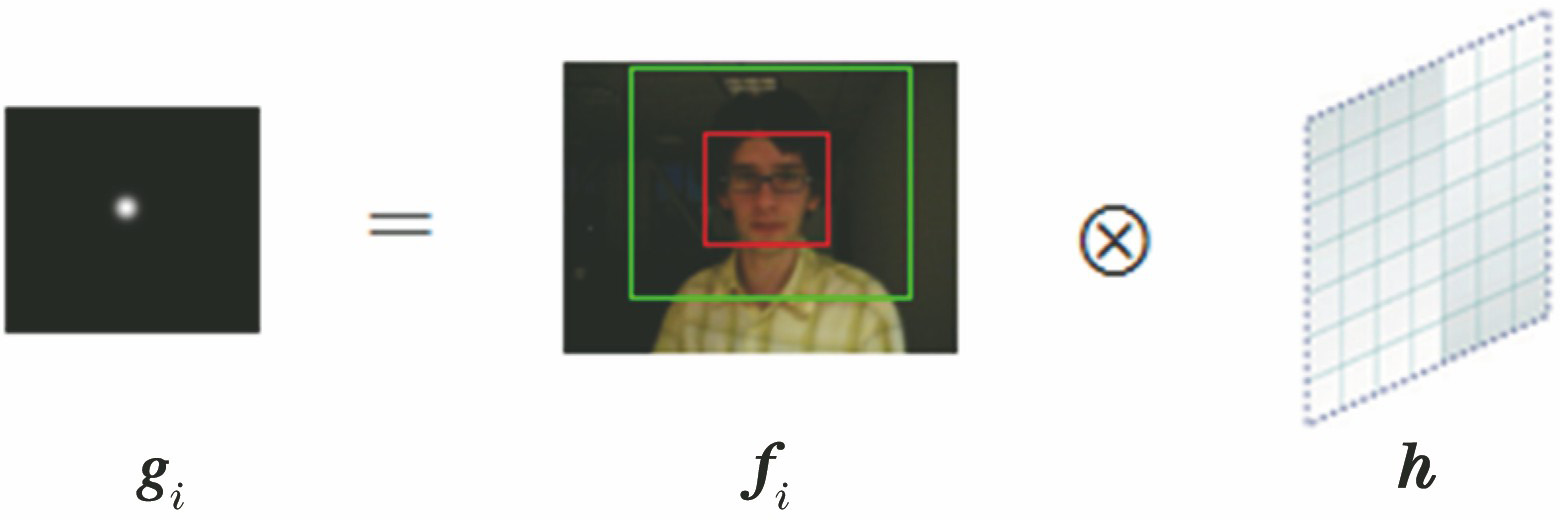
Fig. 1. Application of correlation filtering algorithm in visual tracking
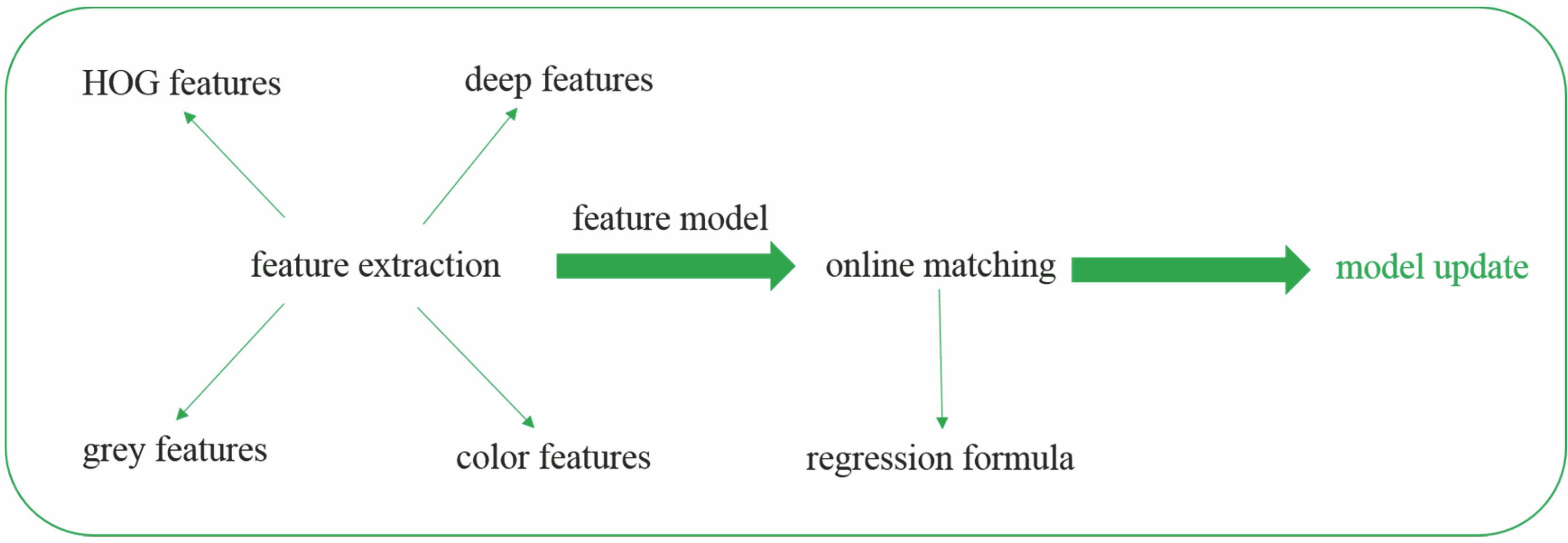
Fig. 2. Flow chart of the correlation filtering target tracking algorithm
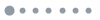
Fig. 3. Tracking effect with a learning rate of 0.01
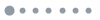
Fig. 4. Tracking effect with a learning rate of 0.05
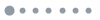
Fig. 5. Response graph and maximum response value when tracking is good. (a) Response graph; (b) tracking effect graph
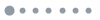
Fig. 6. Response graph and maximum response value when tracking is difficult. (a) Response graph; (b) tracking effect graph
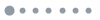
Fig. 7. Response graph and APCE value when tracking is good. (a) Response graph; (b) tracking effect graph
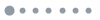
Fig. 8. Response graph and APCE value when tracking is difficult. (a) Response graph; (b) tracking effect graph
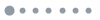
Fig. 9. Tracking results of fast motion objects by different algorithms. (a) Fixed model; (b) adaptive model
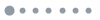
Fig. 10. OPE curves of different algorithms. (a) Average precision; (b) success rate
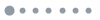
Fig. 11. OPE curves of different algorithms in fast motion target sequences. (a) Average precision; (b) success rate
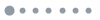
Fig. 12. OPE curves of different algorithms in the motion blur target sequence. (a) Average precision; (b) success rate
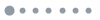
Fig. 13. OPE curves of different algorithms in the rapid deformation target sequences. (a) Average precision; (b) success rate
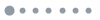
Fig. 14. Test results of our algorithm on the skier data set
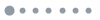
Fig. 15. Verification result of the recheck mechanism. (a) Target is blurred; (b) target is deformed; (c) poor continuity; (d) dislocation tracking
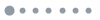
Fig. 16. OPE curve of our algorithm on the self-made data set. (a) Average precision; (b) success rate
|
Table 1. Performance analysis of different algorithms
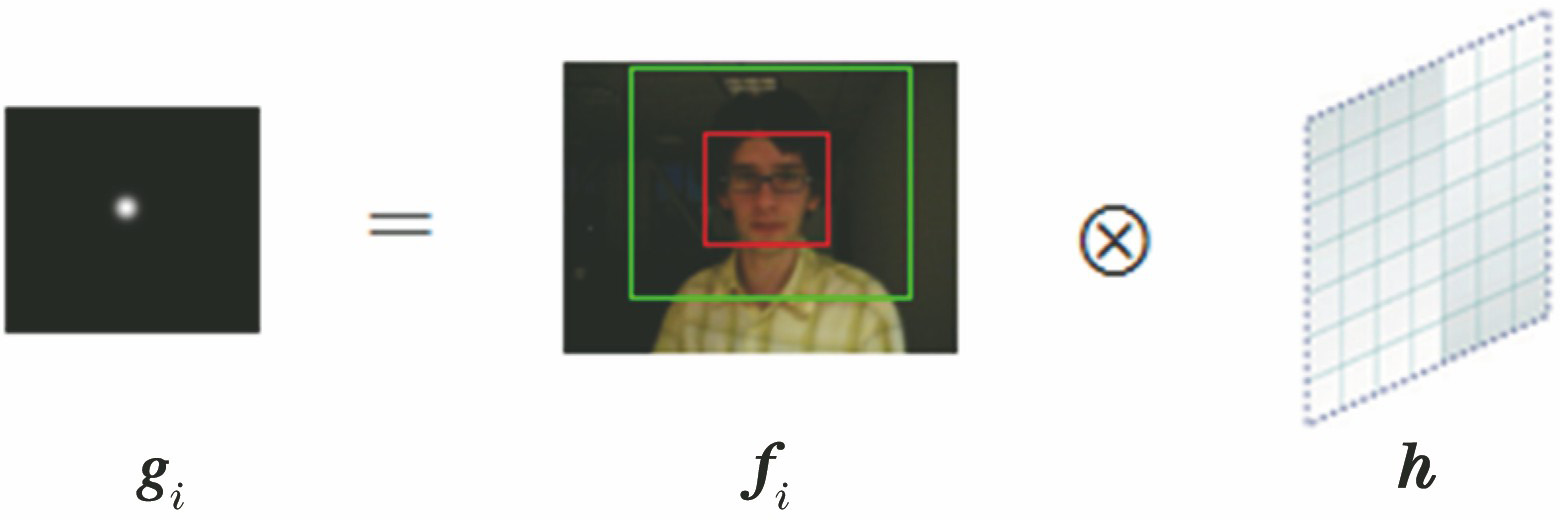
Set citation alerts for the article
Please enter your email address