
- Journal of Geographical Sciences
- Vol. 30, Issue 10, 1702 (2020)
Abstract
Keywords
1 Introduction
Fundamental changes have taken place in the structure of the international system since the end of the Cold War; moreover, changes in the mutual interest and demand between the three major powers-the United States, Russia and China, have resulted in fundamental changes to the nature of their interactions (
Along with the emergence of big data and computational social science (
Scholars have used event data to analyze interstate interactions for decades. However, most of the existing quantitative literature that uses event data to analyze the interactions between the three countries is focused on the Cold War period. Manually coded event datasets of the Conflict and Peace Data Bank (COPDAB), available from 1948 to 1978 (
The interactions between the USA, Russia and China after the end of the Cold War are fundamentally different from those during the Cold War period (
Therefore, our objective is to investigate the cooperative and conflictual interactions between the USA, Russia and China using the temporal scales of event data from GDELT from 1992 to 2016, aiming at providing a quantitative insight into the interactions between the three dyads. Specifically, we utilize twelve monthly-aggregated variables (six cooperative and six conflictual variables) between the three countries obtained from the GDELT and the CCDF method and the VAR model to study their direct cooperative and conflictual interactions between the three dyads, including analyzing the frequencies and intensities of their interactions, the differences in the degree of their cooperative and conflictual behaviors, as well as the interdependence of their cooperative and conflictual interactions between the three dyads.
The remainder of this paper proceeds as follows. Section 2 briefly introduces our research design, describes the measures of cooperation and conflict data obtained from GDELT, and briefly introduces the CCDF and the VAR method. We present and analyze the results of the CCDF method and the VAR model in Section 3. Lastly, we provide a conclusion and discussion of the results and directions for future work in Section 4.
2 Data and methods
This section includes three parts. First, we illustrate the division of the study period from January, 1992 to December, 2016 based on the occurrence of the terrorist attacks on September 11, 2001. The first period is from January, 1991 to September, 2001, and the second is from October 2001 to December 2016. Second, we describe the event data from GDELT and the constructed measures to represent the cooperative and conflictual behaviors between the three dyads. Lastly, we briefly introduce the CCDF and the VAR method.
2.1 Division of the study period
To explore the temporal characteristics of the three dyads’ interactions, the leadership changes in each country, any major international incidents in which the great powers were involved, and statistical method were generally used to divide the time periods (
2.2 Data source and measures of cooperation and conflict
The data used in this paper ranging from January 1992 to December 2016 were obtained from GDELT (
Dyads | Direction | Variables | Descriptions of variables |
---|---|---|---|
USA-CHN dyad | USA-CHN | Scales of cooperative/conflictual behavior from the USA toward China | |
CHN-USA | Scales of cooperative/conflictual behavior from China toward the USA | ||
USA-RUS dyad | USA-RUS | Scales of cooperative/conflictual behavior from the USA toward Russia | |
RUS-USA | Scales of cooperative/conflictual behavior from Russia toward the USA | ||
RUS-CHN dyad | RUS-CHN | Scales of cooperative/conflictual behavior from Russia toward China | |
RUS-CHN | Scales of cooperative/conflictual behavior from China toward Russia |
Table 1.
Descriptions of the monthly time-series variables
There are several advantages of using GDELT data. First, the time range of events in GDELT spans from 1979 to the present and covers our research period. The temporal resolution of events is daily and thus allows to be monthly-aggregated to capture interactions between countries, but some bilateral data (e.g., United Nations Assembly votes) can only provide annual data. Second, GDELT collects news data from extensive sources in more than 65 languages from all over the world, and thus help to avoid the problem of selective bias. For example,
The reasons for adopting monthly-aggregated data as the unit of analysis are as follows. Noise will be included in daily-aggregated or weekly-aggregated data (
2.3 Methods
The CCDF method has been introduced to estimate distributions and examine the differences in the degree of cooperative and conflictual behaviors between the USA and China (
The VAR model is applied to analyze the interdependence of the cooperative and conflictual interactions between the three dyads. The VAR model was initially proposed by
where
Before performing the VAR model, it is necessary to explore the stationarity of time-series variables. A commonly used method—the Augmented Dickey-Fuller (ADF) test (
3 Results
3.1 The frequencies and intensities of the interactions
To understand the overall characteristics of the cooperative and conflictual interactions between the dyads of USA-China, USA-Russia and Russia-China, we briefly analyze the frequencies and intensities of the cooperative and conflictual interactions as indicated by the summed-up amounts and summed-up scales of events between the three dyads. The total numbers and scales of cooperation and conflict between the three dyads are displayed in
In the period from 1992.01 to 2001.09, as shown in Figures 1a and 1b, it can be seen that the amounts and absolute values of scales of cooperation and conflict between the USA and Russia were both highest, followed by the those between the USA and China, while those between Russia and China were the least in the three dyads. However, the frequencies and intensities of the interactions between the USA and China, as indicated by the numbers and absolute scales in 2001.10-2016.12 displayed in Figures 1c and 1d, slightly exceeded those between the USA and Russia to become dominant in the three dyad interactions. This result indicated that China received more attention due to its growing strength, especially China’s GDP went up from the 6th in 2001 to the 2nd in 2016 in the world, and China’s ranking in goods trade rose from the fourth-largest in 2001 to the second-largest country in 2016.
Figure 1.
In both periods, despite prevailing cooperation, there were expanded conflict between the three dyads, as indicated by that the total numbers and absolute scales of cooperation were higher than those of conflict in two periods and the ratio of the conflictual interactions to the cooperative interactions in three dyads in Figures 1b and 1d became larger than their corresponding values in the first period in Figures 1a and 1c. However, the ratio in the dyad of USA-Russia and USA-China were higher than those in the dyad of Russia-China, indicating that conflict in the dyad of USA-Russia and USA-China were stronger than those between Russia and China. In the dyad of USA-Russia in the second period, this ratio increased from 33% to 59% and became the largest ratio, indicating that the conflictual interactions between the USA and Russia intensified, this is mainly related to their geostrategic contradiction, especially in Georgia, Syria and Ukraine, as shown by the troughs and spikes in September 2008, September 2013, March and April 2014 in
Figure 2.
3.2 Differences in the degree of cooperative and conflictual behaviors
This section will analyze the differences in the degree of cooperative and conflictual behaviors between the three dyads using the CCDF technique. To reliably estimate the distributions of the event scales data with wide-range, we both took logarithm of the cooperative scales and the absolute conflictual scales and then estimated their CCDF, according to the practice in
In the dyad of the USA-China (
Figure 3.
In the USA-Russia dyad (
Figure 4.
In the dyad of Russia-China, the CCDF curves of cooperative scales were similar in Figures 5a and 5c, suggesting that a similar probability of cooperative behaviors of Russia and China toward each other in both periods. While the CCDF curves of conflictual scales from Russia to China were both higher than those from China to Russia in the ranges of 1.5 to 3 and 2 to 4 in Figures 5b and 5d, and had higher scales of conflict as illustrated by the long tail in Figures 5b and 5d. Those differences indicated that the conflictual behaviors of sent from Russia toward China were stronger than those from China toward Russia. Thus, it was interesting to see that Russia had been consistently giving China a hard time when dealing with conflict in both periods.
Figure 5.
3.3 Interdependence of cooperative and conflictual interactions
This section would examine and discuss the interdependence of cooperative and conflictual interactions between the three dyads based on the results of VAR models. The significance level of the coefficients of the VAR models listed in Tables 2-4 illustrates how different inferences of the causality of the interactions. The notations of ***, **, and * indicate that the variables are significant at the statistical level of 5%, 10%, and 20%, respectively. According to the suggestion of
From the significance level of the Granger/Wald test in
Independent | 1992.01-2001.09 period | 2001.10-2016.12 period | ||||||
---|---|---|---|---|---|---|---|---|
Dependent variable | Dependent variable | |||||||
- | 0.038*** | 0.091** | 0.159* | - | 0.767 | 0.681 | 0.615 | |
0.649 | - | 0.090** | 0.389 | 0.572 | - | 0.306 | 0.234 | |
0.296 | 0.460 | 0.161* | 0.543 | 0.052** | 0.033*** | 0.054** | 0.571 | |
0.264 | 0.383 | 0.208 | 0.849 | 0.156* | 0.146* | 0.224 | 0.633 | |
0.007*** | 0.019*** | 0.328 | 0.024*** | 0.444 | 0.342 | 0.503 | 0.619 | |
0.007*** | 0.018*** | 0.382 | 0.018*** | 0.432 | 0.331 | 0.319 | 0.646 | |
0.866 | 0.754 | - | 0.149* | 0.027*** | 0.031* | - | 0.010*** | |
0.295 | 0.537 | 0.042*** | - | 0.760 | 0.698 | 0.269 | - | |
0.143* | 0.210 | 0.146* | 0.012*** | 0.833 | 0.836 | 0.083** | 0.078** | |
0.872 | 0.873 | 0.933 | 0.867 | 0.856 | 0.979 | 0.101* | 0.550 | |
0.223 | 0.485 | 0.357 | 0.880 | 0.127* | 0.055** | 0.283 | 0.671 | |
0.473 | 0.561 | 0.496 | 0.669 | 0.557 | 0.253 | 0.829 | 0.731 | |
0.726 | 0.713 | 0.558 | 0.570 | 0.892 | 0.897 | 0.912 | 0.914 | |
Adjusted | 0.694 | 0.680 | 0.506 | 0.519 | 0.884 | 0.890 | 0.905 | 0.908 |
22.741 | 21.320 | 10.826 | 11.356 | 115.926 | 122.708 | 145.113 | 149.057 |
Table 2.
The significance level of the Granger/Wald test of the USA-China dyad in both periods
From the significance level of the USA-Russia dyad in
Independent variables | 1992.01-2001.09 period | 2001.10-2016.12 period | ||||||
---|---|---|---|---|---|---|---|---|
Dependent variable | Dependent variable | |||||||
0.759 | 0.642 | 0.431 | 0.190* | 0.097 | 0.060** | 0.678 | 0.720 | |
0.842 | 0.719 | 0.568 | 0.273 | 0.055** | 0.032*** | 0.642 | 0.840 | |
- | 0.579 | 0.304 | 0.123* | - | 0.827 | 0.762 | 0.900 | |
0.112* | - | 0.163* | 0.113* | 0.856 | - | 0.805 | 0.766 | |
0.518 | 0.388 | 0.504 | 0.753 | 0.930 | 0.862 | 0.887 | 0.916 | |
0.250 | 0.166* | 0.338 | 0.535 | 0.944 | 0.878 | 0.927 | 0.938 | |
0.832 | 0.770 | 0.656 | 0.919 | 0.057** | 0.049*** | 0.308 | 0.208 | |
0.651 | 0.387 | 0.796 | 0.878 | 0.937 | 0.720 | 0.460 | 0.644 | |
0.391 | 0.626 | - | 0.332 | 0.123* | 0.159* | - | 0.003*** | |
0.863 | 0.559 | 0.511 | - | 0.430 | 0.287 | 0.787 | - | |
0.969 | 0.958 | 0.723 | 0.585 | 0.416 | 0.470 | 0.346 | 0.675 | |
0.978 | 0.941 | 0.542 | 0.605 | 0.604 | 0.428 | 0.886 | 0.535 | |
0.553 | 0.540 | 0.597 | 0.604 | 0.869 | 0.869 | 0.880 | 0.849 | |
Adjusted | 0.501 | 0.486 | 0.550 | 0.558 | 0.860 | 0.860 | 0.872 | 0.839 |
10.628 | 10.075 | 12.707 | 13.093 | 93.769 | 93.501 | 103.572 | 79.374 |
Table 3.
The significance level of the Granger/Wald test of the USA-Russia dyad in both periods
In the dyad of Russia and China as shown in
Generally, there were three-quarters of the 12 cooperative variables (dependent variables), which were Granger caused by conflictual variables, and 7 of 12 conflictual variables (dependent variables), which were Granger caused by cooperative variables. This result supported our specification that incorporating the cooperative and conflictual variables into the VAR models, and suggesting the interdependence between the cooperative behaviors and the conflictual behaviors between the three dyads.
Independent variables | 1992.01-2001.09 period | 2001.10-2016.12 period | ||||||
---|---|---|---|---|---|---|---|---|
Dependent variable | Dependent variable | |||||||
0.919 | 0.795 | 0.950 | 0.553 | 0.445 | 0.446 | 0.613 | 0.339 | |
0.673 | 0.807 | 0.626 | 0.728 | 0.236 | 0.263 | 0.856 | 0.538 | |
0.627 | 0.502 | 0.598 | 0.091 | 0.011*** | 0.011*** | 0.312 | 0.172* | |
0.732 | 0.681 | 0.914 | 0.044*** | 0.007*** | 0.009*** | 0.277 | 0.163* | |
- | 0.601 | 0.911 | 0.119* | - | 0.845 | 0.745 | 0.799 | |
0.480 | - | 0.971 | 0.415 | 0.696 | - | 0.851 | 0.603 | |
0.006*** | 0.001*** | 0.973 | 0.608 | 0.514 | 0.661 | 0.581 | 0.777 | |
0.004*** | 0.001*** | 0.614 | 0.602 | 0.303 | 0.398 | 0.359 | 0.195* | |
0.965 | 0.453 | 0.131* | 0.048*** | 0.062** | 0.153* | 0.028*** | 0.195* | |
0.745 | 0.670 | 0.187* | 0.905 | 0.566 | 0.833 | 0.181* | 0.360 | |
0.000*** | 0.000*** | - | 0.887 | 0.272 | 0.214 | - | 0.198* | |
0.001*** | 0.000*** | 0.054** | - | 0.184* | 0.202 | 0.917 | - | |
0.539 | 0.561 | 0.444 | 0.454 | 0.692 | 0.684 | 0.682 | 0.695 | |
Adj. | 0.485 | 0.509 | 0.379 | 0.391 | 0.670 | 0.662 | 0.659 | 0.673 |
10.024 | 10.947 | 6.845 | 7.145 | 31.616 | 30.524 | 30.180 | 32.109 |
Table 4.
The significance level of the Granger/Wald test of China-Russia dyad in both periods
4 Discussion and conclusions
This analysis used cooperative and conflictual scales of event data from GDELT and statistical methods to quantitatively investigate the interactions between the USA, Russia and China after the end of the Cold War. We analyzed the variations in the frequencies and intensities of their cooperative and conflictual interactions, differences in the degree of their cooperative and conflictual behaviors using the CCDF technique, as well as the interdependence of their cooperative and conflictual behaviors using the VAR model between the three dyads. Our results provided quantitative insights into the direct interactions between the three dyads and helps us to understand their interactions better in the post-Cold War period. The results are as follows.
The frequencies and strengths of the USA-Russia interactions ranked first in the first period, while the interactions between the USA-China became the dominant interactions in the second period. The Russia-China interactions always occupied the third place. In the two periods, cooperation prevailed in the three dyads, but the conflictual interactions in the dyads of the USA-Russia and the USA-China were strong, especially the conflictual interactions between the USA and Russia tended to more intense in the second period, mainly related to their strategic contradiction in Georgia, Ukraine and Syria.
The USA and China took similar cooperative actions toward each other suggested by a similar pattern of CCDF curves in the first period, while the USA had a relatively higher probability of sending cooperative behaviors to China due to the increasingly extensive cooperation sent from the USA to China since 9/11 in 2001. The USA and China may take similar actions toward each other in both periods when dealing with conflict, indicated by a similar CCDF pattern of conflictual scales. The USA and Russia, as well as Russia and China, both took similar cooperative behaviors toward each other, as indicated by the approximately overlapping CCDF curves of cooperative scales between the two dyads. However, there were differences in conflictual behaviors toward each other in the two dyads: the conflictual behaviors in the USA-Russia dyads showed complicated characteristic, and Russia had been consistently giving China a hard time in both periods when dealing with conflict.
The VAR results suggested the interdependence between cooperative and the conflictual behaviors in the three dyads, as shown that three-quarters of the 12 cooperative variables (dependent variables) were Granger caused by conflictual variables, and 7 of 12 conflitual variables (dependent variables) were Granger caused by cooperative variables. In the three dyads, Russia’s behaviors toward the two countries always Granger caused their cooperative interactions between the USA and China, and the conflict sent from the USA to Russia always Granger caused its conflictual behaviors to China. The VAR results indicated that China played a limited role in the interactions of the USA-Russia for that the behaviors of China only Granger caused the cooperation between the USA and Russia, but it had never been the Granger cause of their conflictual interactions in both periods. These results quantitatively supported the asymmetrical interdependent interaction patterns between the USA and China, the USA and Russia, as well as Russia and China.
The main objective of our analysis is to investigate the directly cooperative and conflictual interactions between the three dyads from the time-series perspective since the end of the Cold War. Therefore, as for understanding what factors affect the interactions between the three dyads, we would briefly discuss the potential factors, including military strength, economic strength, economic independence (especially trade independence), geopolitical factors and others (
Besides these results, we also would like to discuss how the GDELT event data is used in quantifying the behaviors and interactions between countries and help to provide references for researchers. In the existing studies, event data from GDELT to measure the interactions between dyads is used in four ways: (1) temporally aggregated net scales of events (
Some limitations are to be illustrated. How other countries affect the interactions between the three countries from the international political network was not provided. In the future, we will attempt to investigate other countries’ impacts on the three countries’ interactions using the social network method. GDELT database also provides coordinate information (i.e., latitude and longitude) for events and thus make it possible to utilize methods of spatial analysis to investigate the spatial distribution and dynamics of the different types of interactions between the three countries, and identify “hot” locations with intense conflicts to enhance understanding about the spatial pattern of the geopolitical conflicts. GDELT data with coordinate information provides geographers with the potential to investigate questions related to geopolitics and political geography proposed by several domestic scholars (
Declaration
The views of this paper do not represent the views of the project.
Acknowledgement
We would like to thank the high-performance computing support from the Center for Geodata and Analysis, Faculty of Geographical Science, Beijing Normal University [
References
[1] AbbP, StrüverG. Regional linkages and global policy alignment: The case of China-Southeast Asia relations. In: German Institute of Global and Area Studies(2015).
[2] Azar EE. The Conflict and Peace Data Bank (COPDAB) Project. Journal of Conflict Resolution, 24, 143-152(1980).
[3] Bodas-Sagi DJ, Labeaga JM. Using GDELT data to evaluate the confidence on the Spanish government energy policy. International Journal of Interactive Multimedia and Artificial Intelligence, 3, 38-43(2016).
[4] ChenXiaoqiang, YuanLihua, ShenShi et al. The analysis of geo-relationships among China and its neighboring countries. Acta Geographica Sinica, 74, 1534-1547(2019).
[5] ChiZhipei, HouNa. Quantitative research on big data and bilateral relations: A case study of GDELT and Sino-US relations. Quarterly Journal of International Politics, 4, 67-88(2019).
[6] CukierK, Mayer-SchoenbergerV. The rise of big data: How it’s changing the way we think about the world. Foreign Affairs, 92, 28-41(2013).
[7] Dickey DA, Fuller WA. Distribution of the estimators for autoregressive time series with a unit root. Journal of the American statistical Association, 74, 427-431(1979).
[8] Dickey DA, Fuller WA. Likelihood ratio statistics for autoregressive time series with a unit root. Econometrica, 49, 1057-1072(1981).
[9] Dixon WJ. Reciprocity in United States-Soviet relations: Multiple symmetry or issue linkage?. American Journal of Political Science, 30, 421-445(1986).
[10] DuDebin, DuanDezhong, LiuChengliang et al. Twenty-five years of progress in geopolitics research: Efforts from China's geographers. Journal of Geographical Sciences, 26, 1223-1242(2016).
[11] ElshendyM, FronzettiC. Big data analysis of economic news: Hints to forecast macroeconomic indicators. International Journal of Engineering Business Management, 9, 1-12(2017).
[12] Freeman JR, Williams JT, LinT. Vector autoregression and the study of politics. American Journal of Political Science, 33, 842-877(1989).
[13] Gao JB, HuJ. Financial crisis, Omori’s law, and negative entropy flow. International Review of Financial Analysis, 33, 79-86(2014).
[14] Gao JB, Leetaru KH, Hu J et al. Massive media event data analysis to assess world-wide political conflict and instability. In: Greenberg A M, Kennedy W G, Bos N D, 2013. Social Computing, Behavioral-Cultural Modeling and Prediction, 284-292(2013).
[15] GeQuansheng, JiangDong, LuFeng et al. Spatio-temporal simulation of the geopolitical environment system. Journal of Geographical Sciences, 28, 871-880(2018).
[16] GladkyyO. American foreign policy and US relations with Russia and China after 11 September. World Affairs, 166, 3-23(2003).
[17] Goldstein JS. Reciprocity in superpower relations: An empirical analysis. International Studies Quarterly, 35, 195-209(1991).
[18] Goldstein JS. A conflict-cooperation scale for WEIS events data. Journal of Conflict Resolution, 36, 369-385(1992).
[19] Goldstein JS, Freeman JR. U.S.-Soviet-Chinese relations: Routine, reciprocity, or rational expectations?. American Political Science Review, 85, 17-35(1991).
[20] Gonzalo D CS, GarcíaherreroA, OrtizA et al. An empirical assessment of social unrest dynamics and state response in Eurasian countries. Social Science Electronic Publishing, 3, 1-29(2015).
[21] GuoZhenyuan. China-US-Russia relationship since September 11. Peace and Development, 1-5(2002).
[22] Kalyvas SN, BalcellsL. International system and technologies of rebellion: How the end of the cold war shaped internal conflict. American Political Science Review, 104, 415-429(2010).
[23] KangH, ReuvenyR. An exploration of multi-country political conflict/cooperation interdependence. International Interactions, 26, 129-152(2000).
[24] KangH, ReuvenyR. Exploring multi-country dynamic relations between trade and conflict. Defence & Peace Economics, 12, 175-196(2001).
[25] Kornberg JF. Chinese foreign policy: Theory and practice (review). China Review International, 3, 251-254(1996).
[26] LazerD, PentlandA, AdamicL et al. Computational social science. Science, 323, 721-723(2014).
[27] LeetaruK, Schrodt PA. GDELT: Global data on events, location, and tone, 1979-2012. In: The International Studies Association. Annual Conference of the International Studies Association. San Diego, California, March, 29, 2013(2013).
[28] LevinN, AliS, CrandallD. Utilizing remote sensing and big data to quantify conflict intensity: The Arab Spring as a case study. Applied Geography, 94, 1-17(2018).
[29] Li PW. The role of USA in Cross-Strait relations: A time series analysis approach. Soochow Journal Political Science., 33, 207-270(2015).
[30] LiQingsi. The Sino-US-Russian relations in dynamic balance. Frontiers, 6-14(2017).
[31] LiSaojun. Conflict-cooperation model: Quantitative analysis of Sino-US relations. World Economics and Politics, 43-49(2002).
[32] LiXing, KongRui. Russian factors on Sino-US relationship. Russian, East European & Central Asian Studies, 60-68(2010).
[33] LiuFenghua. Triangular relationship among China, Russia and the US after the Ukraine crisis: Adjustment and trend. International Economic Review, 103-115(2017).
[34] LiuYi, WangYun, YangYu et al. Regional integration and interaction of the Guangdong-Hong Kong-Macao Greater Bay Area. Acta Geographica Sinica, 74, 2455-2466(2019).
[36] LuM. Vector Autoregression (VAR): An approach to dynamic analysis of geographic processes. Geografiska Annaler. Series B, Human Geography, 83, 67-78(2001).
[37] MaMenglan, FangPeng, Gao Jianbo et al. Does ideology affect the tone of international news coverage? In: 2017 International Conference on Behavioral, Economic, Socio-Cultural Computing (BESC)(2017).
[38] MaMingqing, YuanWu, GeQuansheng et al. Big data analysis of social development situation in regions along the Belt and Road. Progress in Geography, 38, 1009-1020(2019).
[39] McGinnis MD, Williams M GT. Change and stability in superpower rivalry. The American Political Science Review, 83, 1101-1123(1989).
[40] Moore WH. Action-reaction or rational expectations? Reciprocity and the domestic-international conflict nexus during the "Rhodesia Problem". Journal of Conflict Resolution, 39, 129-167(1995).
[41] PangXun, LiuZiye. China-U.S. in Massive Machine-coded event data: Influence of reciprocity, policy inertia, and a third power. World Economics and Politics, 53-79(2019).
[42] QiHaixia. An analysis of the factors affecting China’s relations with the other major powers. Chinese Journal of European Studies, 61-78(2012).
[43] RajmairaS, Ward R MD. Evolving foreign policy norms: Reciprocity in the superpower triad. International Studies Quarterly, 34, 457-475(1990).
[44] ShenShi, SongChangqing, ChengChangxiu et al. GDELT: Big event data for sensing global social dynamics. World Regional Studies, 29, 71-76(2020).
[45] ShenShi, YuanLihua, YeSijing et al. The fluctuation and background analysis of geopolitical relations between China and USA during the last 40 years. Scientia Geographica Sinica, 39, 1063-1071(2019).
[46] SimsC. A comparison of interwar and postwar business cycles: Monetarism reconsidered. American Economic Review, 70, 250-257(1980).
[47] SongChangqing, GeYuejing, LiuYungang et al. Under taking research on Belt and Road Initiative from the geo-relation perspective. Geographical Research, 37, 3-19(2018).
[48] Theocharous AL, AnastasiosZ, NeophytosL et al. Tourism, instability and regional interdependency: Evidence from the Eastern-Mediterranean. Defence and Peace Economics, 31, 245-268(2018).
[49] Ward MD. Cooperation and conflict in foreign policy behavior: Reaction and memory. International Studies Quarterly, 26, 87-126(1982).
[50] Ward MD, RajmairaS. Reciprocity and norms in U.S.-Soviet foreign policy. Journal of Conflict Resolution, 36, 342-368(1992).
[51] Weidmann NB. A closer look at reporting bias in conflict event data. American Journal of Political Science, 60, 206-218(2016).
[52] YanXuetong. The instability of China-US relations. The Chinese Journal of International Politics, 3, 263-292(2010).
[53] YanXuetong, QiHaixia. Football game rather than boxing match: China-US intensifying rivalry does not amount to Cold War. The Chinese Journal of International Politics, 5, 105-127(2012).
[54] Yonamine JE. A nuanced study of political conflict using the Global Datasets of Events Location and Tone (GDELT) dataset. University Park, Pennsylvania: Doctoral Dissertation of Pennsylvania State University, University Park, 1-183(2013).
[55] YuanYihong, LiuYu, WeiGuixing. Exploring inter-country connection in mass media: A case study of China. Computers Environment & Urban Systems, 62, 86-96(2017).
[57] ZhaoHuasheng. Research on the New Triangular Relationship between China, Russia and the U.S. Russian, Central Asian & East European Studies, 5-29(2018).
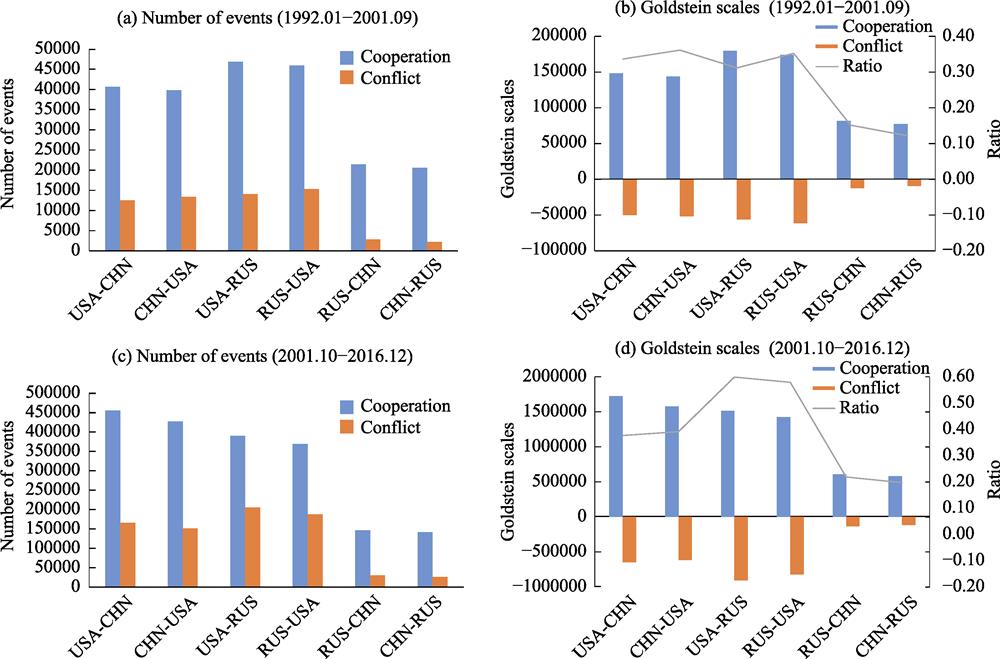
Set citation alerts for the article
Please enter your email address