LIANG Liang, TANG Shou-feng, TONG Min-ming, DONG Hai-bo. Study on the Detection Method of the Granularity of Pulverized Coal Based on THz Time-Domain Chaos Features[J]. Spectroscopy and Spectral Analysis, 2019, 39(5): 1392

Search by keywords or author
- Spectroscopy and Spectral Analysis
- Vol. 39, Issue 5, 1392 (2019)
Abstract
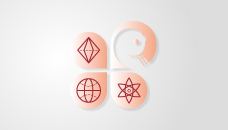
Set citation alerts for the article
Please enter your email address