Ying Li, Jianglei Di, Li Ren, Jianlin Zhao, "Deep-learning-based prediction of living cells mitosis via quantitative phase microscopy," Chin. Opt. Lett. 19, 051701 (2021)

Search by keywords or author
- Chinese Optics Letters
- Vol. 19, Issue 5, 051701 (2021)
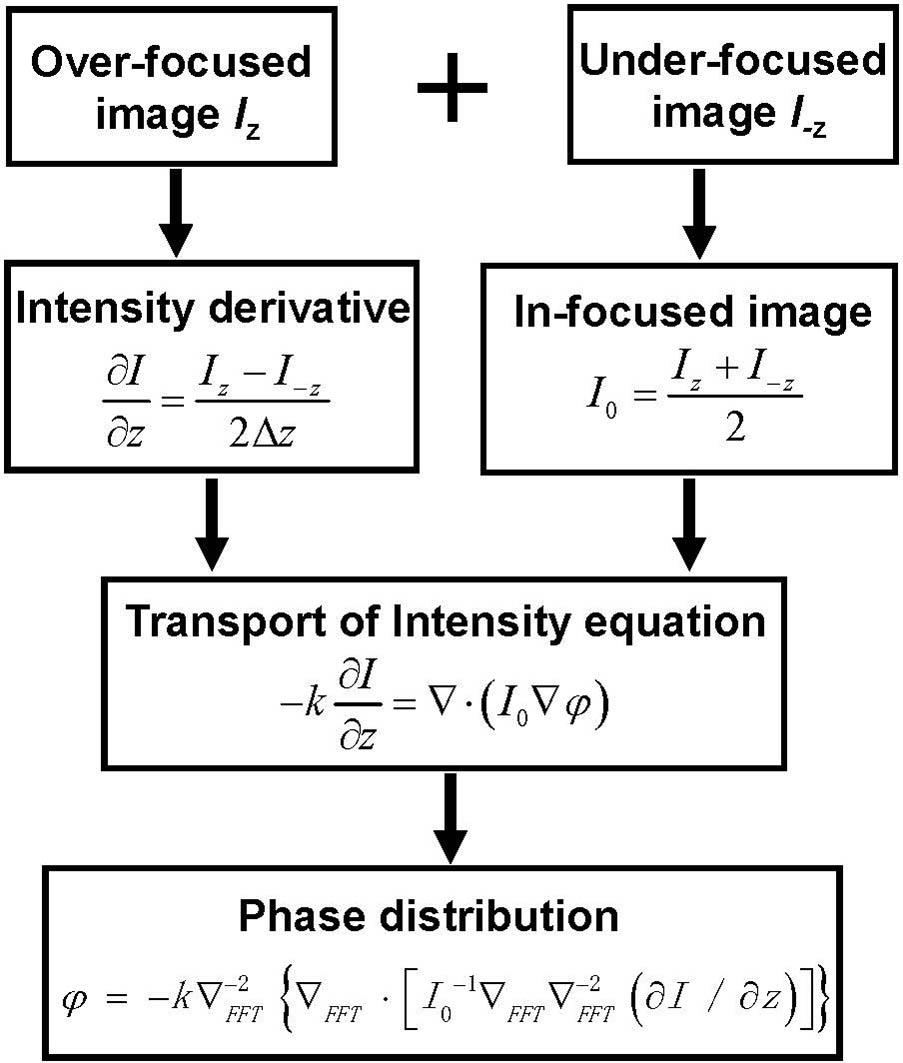
Fig. 1. Flowchart of algorithm for QPM based on the TIE.
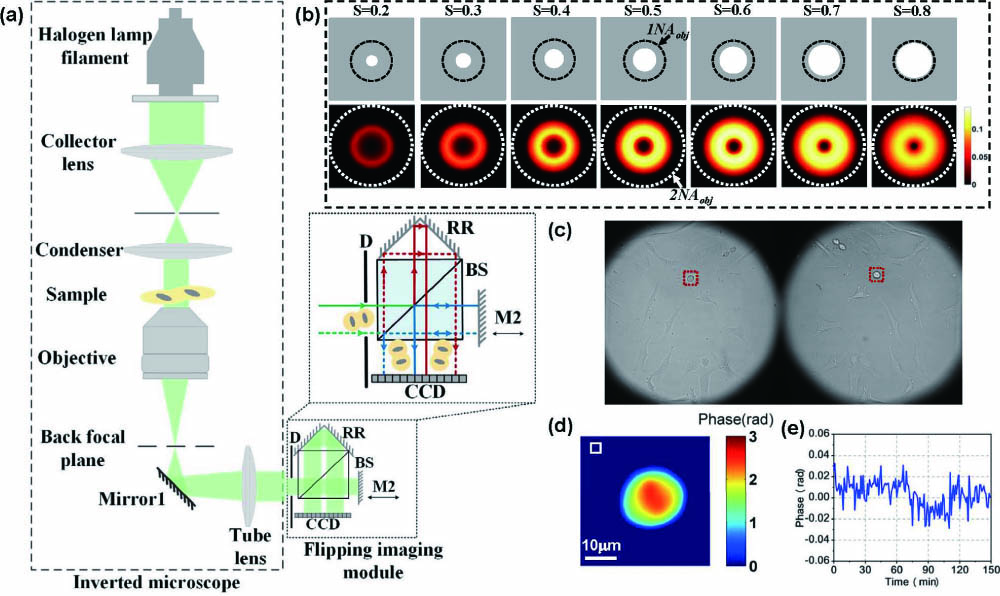
Fig. 2. (a) Experimental setup. D, diaphragm; BS, beam splitter; RR, retroreflector; M2, mirror 2. (b) 2D images of source pattern and corresponding phase transfer function for different coherence parameters. (c) Original lateral-flipped images in single shot. (d) Recovered phase map of the cell marked in the red box in (c). (e) Phase stability measurement during the 150 min experiment in the white box marked in (d).
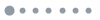
Fig. 3. (a), (b) Representative phase and corresponding intensity images of mitosis. (c), (d) Representative phase and corresponding intensity images of non-mitosis.
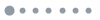
Fig. 4. Workflow diagram and detailed architecture of DCNN for classification of mitosis and non-mitosis.
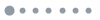
Fig. 5. Learning process of DCNN trained by phase and intensity images, respectively.
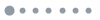
Fig. 6. Performance and t-SNE visualization of the DCNN trained by phase and intensity images, respectively. In t-SNE visualization, number 1 represents mitosis and number 2 represents non-mitosis.
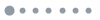
Fig. 7. Overall classification performance for DCNN trained by phase images, DCNN with intensity images and random forest trained by dry mass, respectively. (a) Accuracy. (b) Precision. (c) Recall. (d) F1 score.
|
Table 1. Comparison of Accuracy and F1 Score for Different Classifiers
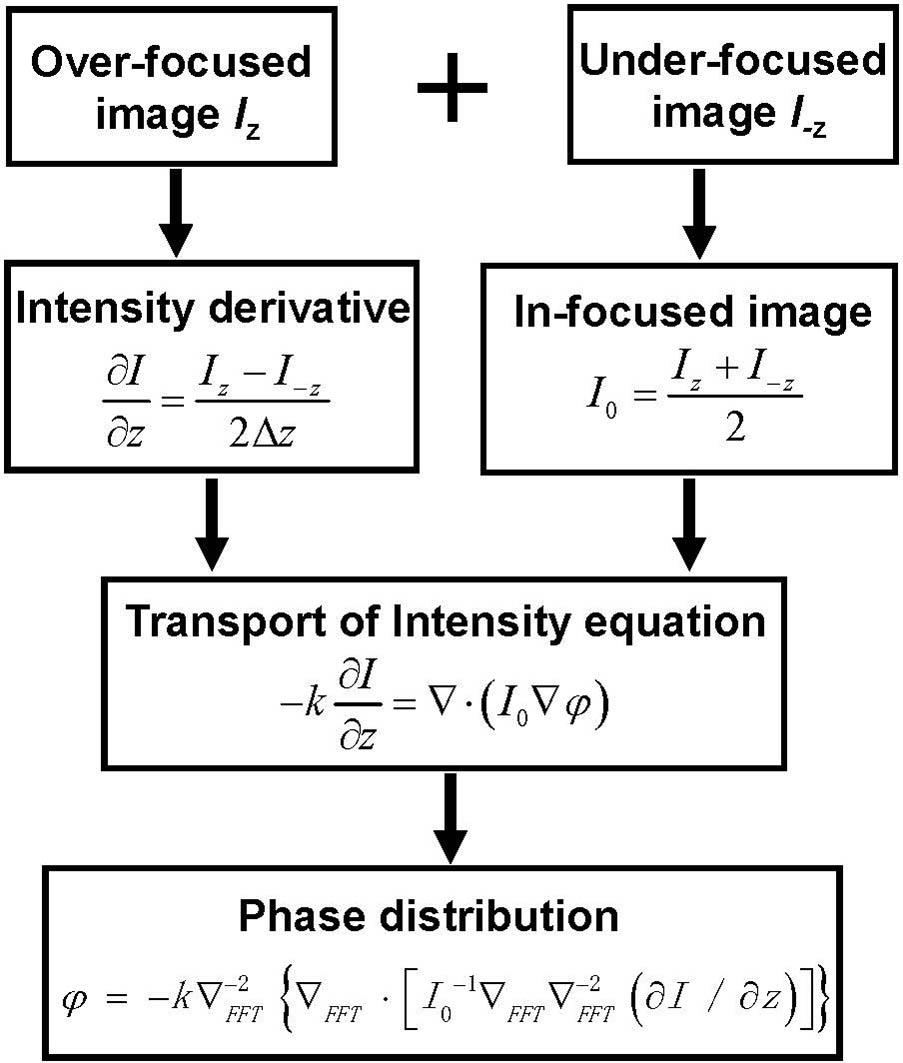
Set citation alerts for the article
Please enter your email address