ZHAO Yong-jian, GUO Rui, WANG Lu-yao, JIANG Bin. Research on Classification of Dwarf Nova Based on Deep Architecture Network[J]. Spectroscopy and Spectral Analysis, 2020, 40(2): 656

Search by keywords or author
- Spectroscopy and Spectral Analysis
- Vol. 40, Issue 2, 656 (2020)
Abstract
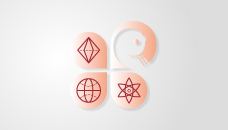
Set citation alerts for the article
Please enter your email address