Yuanxue Xin, Fengting Zhu, Pengfei Shi, Xin Yang, Runkang Zhou. Super-Resolution Reconstruction Algorithm of Images Based on Improved Enhanced Super-Resolution Generative Adversarial Network[J]. Laser & Optoelectronics Progress, 2022, 59(4): 0420002

Search by keywords or author
- Laser & Optoelectronics Progress
- Vol. 59, Issue 4, 0420002 (2022)
Abstract
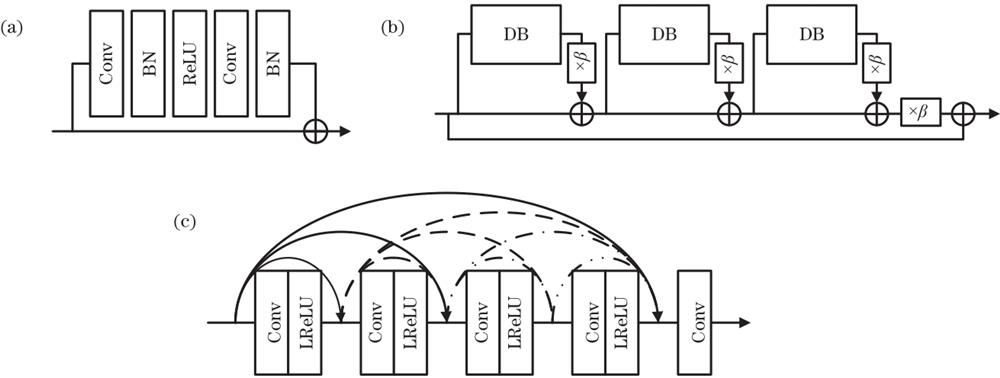
Set citation alerts for the article
Please enter your email address