Yu-hua QIN, Meng ZHANG, Ning YANG, Qiu-fu SHAN. Local Preserving Projection Similarity Measure Method Based on Kernel Mapping and Rank-Order Distance[J]. Spectroscopy and Spectral Analysis, 2021, 41(10): 3117

Search by keywords or author
- Spectroscopy and Spectral Analysis
- Vol. 41, Issue 10, 3117 (2021)
Abstract
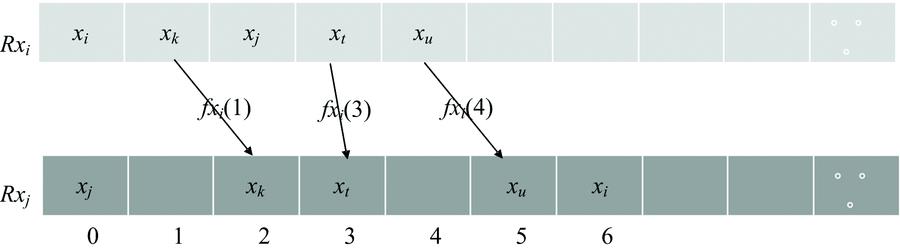
Set citation alerts for the article
Please enter your email address