Jiaxue Wu, Jinbin Gui, Junchang Li, Tai Fu, Wei Cheng. Deep Learning-Based Interference-Free Hologram Generation[J]. Acta Optica Sinica, 2022, 42(14): 1409001

Search by keywords or author
- Acta Optica Sinica
- Vol. 42, Issue 14, 1409001 (2022)
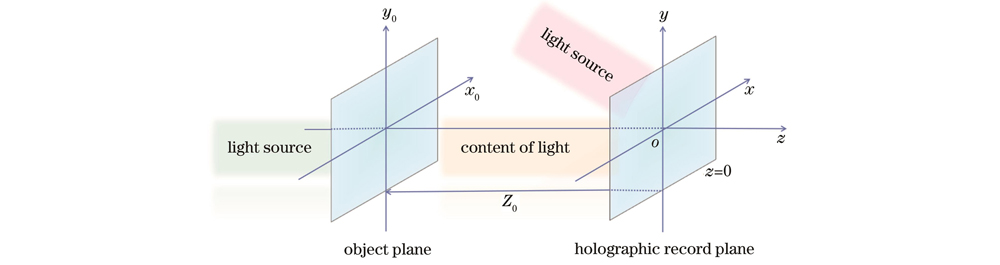
Fig. 1. Schematic diagram of digital hologram recording
![Hologram spectrum and reconstructed images with different sizes of filter windows[11]. (a) Hologram spectrum and filter window; (b) reconstructed image with large size filter window; (c) reconstructed image with small size filter window](/richHtml/gxxb/2022/42/14/1409001/img_02.jpg)
Fig. 2. Hologram spectrum and reconstructed images with different sizes of filter windows[11]. (a) Hologram spectrum and filter window; (b) reconstructed image with large size filter window; (c) reconstructed image with small size filter window
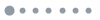
Fig. 3. Deep learning-based interference-free hologram generation network
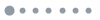
Fig. 4. Simulated data sets. (a1)(a2) Original maps; (b1)(b2) holograms; (c1)(c2) reconstructed images; (d1)(d2) spectrograms
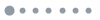
Fig. 5. Simulation experiment data. (a) Original images; (b) holograms; (c) reconstructed images; (d) spectrograms; (e) interference-free holograms; (f) reconstructed images of interference-free holograms
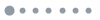
Fig. 6. Actual shooting light path diagram
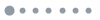
Fig. 7. Actual shooting of Fresnel hologram for verification. (a) Original object; (b) holograms taken actually; (c) spectrogram; (d) interference-free hologram; (e) reconstructed image
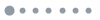
Fig. 8. Loss function error curves. (a) RPN classification loss error curve; (b) RPN regression loss error curve;(c) Fast R-CNN classification loss error curve; (d) Fast R-CNN regression error curve; (e) total training error curve; (f) classification accuracy
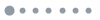
Fig. 9. Experimental procedure for artificial filtering hologram with interference
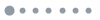
Fig. 10. Images obtained by artificial filtering and HoloZL network. (a) Original images; (b) artificially filtered interference-free holograms; (c) reconstructed images of artificially filtered interference-free holograms; (d) interference-free holograms generated by HoloZL network; (e) reconstructed images of interference-free holograms generated by HoloZL network
|
Table 1. Quality assessment metrics of interference-free hologram
|
Table 2. Quantitative assessment indexes of comparative experiments
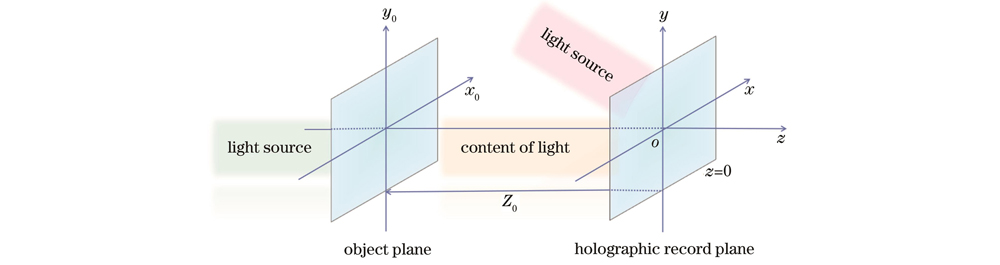
Set citation alerts for the article
Please enter your email address