LI Qing-bo, LI Xiang, ZHANG Guang-jun, XU Yi-zhuang, WU Jin-guang, SUN Xue-jun. Application of Probabilistic Neural Networks Method to Gastric Endoscope Samples Diagnosis Based on FTIR Spectroscopy[J]. Spectroscopy and Spectral Analysis, 2009, 29(6): 1553

Search by keywords or author
- Spectroscopy and Spectral Analysis
- Vol. 29, Issue 6, 1553 (2009)
Abstract
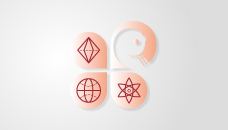
Set citation alerts for the article
Please enter your email address