JIA Wen-shen, ZHANG Heng-zhi, MA Jie, LIANG Gang, WANG Ji-hua, LIU Xin. Study on the Predication Modeling of COD for Water Based on UV-VIS Spectroscopy and CNN Algorithm of Deep Learning[J]. Spectroscopy and Spectral Analysis, 2020, 40(9): 2981

Search by keywords or author
- Spectroscopy and Spectral Analysis
- Vol. 40, Issue 9, 2981 (2020)
Abstract
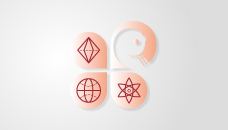
Set citation alerts for the article
Please enter your email address