LI Xin-xing, ZHU Chen-guang, FU Ze-tian, YAN Hai-jun, PENG Yao-qi, ZHENG Yong-jun. Rapid Detection of Soil Moisture Content Based on UAV Multispectral Image[J]. Spectroscopy and Spectral Analysis, 2020, 40(4): 1238

Search by keywords or author
- Spectroscopy and Spectral Analysis
- Vol. 40, Issue 4, 1238 (2020)
Abstract
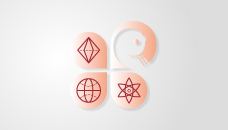
Set citation alerts for the article
Please enter your email address