Wensheng FAN, Fan LIU, Ming LI. Remote Sensing Image Fusion Based on Two-branch U-shaped Transformer[J]. Acta Photonica Sinica, 2023, 52(4): 0428002

Search by keywords or author
- Acta Photonica Sinica
- Vol. 52, Issue 4, 0428002 (2023)
Abstract
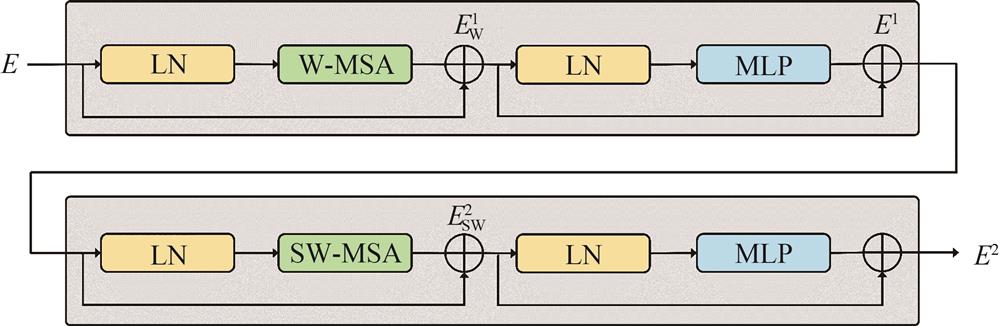
Set citation alerts for the article
Please enter your email address