
- Photonics Research
- Vol. 10, Issue 1, 174 (2022)
Abstract
1. INTRODUCTION
Increasing evidence in neuroscience supports that the neural population plays a critical role in robust information representation, communication, and processing [1]. As an ensemble of neurons and synaptic interconnections, it provides a deeper understanding of the dependence between global functions and elementary details. The neural population features the nonlinear response curve of individual neurons (i.e., bell-shape tuning curves or Gaussian receptive fields) and activities coding at population level [2]. The former reveals that a neuron is interested in a part of the input range and responds to it in a distributed and nonlinear way [3]. The latter indicates population activities constructed by these responses can be regarded as state variables corresponding to input patterns [4]. In recent years, the advances in electronic neuromorphic computing indicate the way for these properties to support population-based information processing [5]. Required nonlinear responses are built up by the weighted sum of a set of superparamagnetic basic functions that agree well with the bell-shape tuning curves [6]. The input vowel patterns can be mapped onto the population activities of four coupled spin-torque nano-oscillators for efficient recognition [7]. Generally, the scalability and interconnection of neurons are prerequisites for accessing a population-based information processing.
However, it remains challenging to achieve a photonic neural population (PNP) equipped with nonlinear response curves and efficient population coding. In neuromorphic photonics, the unique advantages of broad bandwidth and low power consumption have dominated high-speed and massively parallel linear computations (i.e., synaptic weighting) [8]. Numerous studies also focus on the implementations of nonlinear computations (i.e., the neuron activation) based on single devices or temporal nodes, as illustrated in Fig. 1(a) [20]. Specifically, we attribute the challenge of establishing a PNP to the physical complexity, response latency, and reconfigurability. First, the complicated implementation of a single-device-based photonic neuron limits the scalability and interconnection, for example, schemes with a laser [9–14], a modulator [15], and a resonator [16,17]. Second, the scaling up of a temporal photonic neuron introduces unwanted response latency, which may counteract the advantages of a PNP for high-speed processing [18,19]. Third, the reconfigurability of a PNP is critical for broad processing tasks, which is challenging for the photonic neurons based on the embedded nonlinear material with fixed topology [21].
Figure 1.Conceptual illustrations of conventional photonic neurons, biological neural population, and the proposed comb-based photonic neural population (PNP). (a) Existing photonic neurons scale up in the spatial domain or temporal domain at the cost of physical complexity or response latency. The former is based on a single structure or devices, such as lasers [9
Here, we propose a comb-based PNP to enable a population-based scheme for parallel and nonlinear processing. The comparison of the comb-based PNP and its biological counterpart is conceptually illustrated in Figs. 1(b) and 1(c). It is observed that an injected optical frequency comb (OFC) features inverse bell-shape nonlinear responses to input frequency components and that they are reconfigurable through the OFC parameters. Therefore, these distributed responses support a high-dimensional representation of complex input patterns through the comb power distribution (i.e., population activities). It is experimentally indicated that the interconnection between the comb neurons is naturally provided by the carrier coupling. It is found that the lateral inhibition enhances the response nonlinearity and leads to a unique population activity. We also implement a classification task of three input patterns with dual radio-frequency (RF) tones. The distinct population activities of the comb-based PNP can be efficiently read out for classification. For the comb-based PNP, the inherent broad bandwidth of photonics ensures superior scalability and the ability of nonlinear processing of RF input patterns in a time-efficient manner compared to other neuromorphic and conventional photonics-assisted paradigms [22].
Sign up for Photonics Research TOC. Get the latest issue of Photonics Research delivered right to you!Sign up now
2. METHODS
A. Theory
The comb-based PNP includes population generation, parallel processing, and population activity reading out, corresponding to the implementation of a dual-OFC generation, the injected OFC to interact with the input, and a beat process for the dual-OFC, respectively. The scalability and tunability of the OFC [23] help with the flexible control of the PNP properties at both a neuron level (e.g., initial comb power) and a population level (e.g., comb spacing). The monitoring of the population activities benefits from the introduction of another coherent OFC [24] and a beat process. The process is described below. First, the electro-optical dual-OFC is prepared at a comb spacing of
The injection dynamics of an OFC to semiconductor lasers (SLs) dependent on various injection ratios and detuning frequencies was theoretically and experimentally investigated [25,26]. We concentrate on the effect of input frequency on the comb power under different configurations of the OFC and DFB-LD. A theoretical model with a modified term of pump current is used for the numerical simulation (see Appendix A). In the optical cavity, the injected OFC and input frequency act as two sources of carrier modulation to drive the resonance of the output optical field. As a result, they interact with each other through carrier coupling, leading to an input-frequency-dependent variation of the comb power. In numerical investigations, we apply a scanning input frequency and record the comb power to study the shape and width of the response curve, which are related to the DFB-LD bias current, the input RF power, and the initial comb power (see Appendix B). Furthermore, we simulate the responses of 15 comb powers to four complex input patterns with 15 frequency tones to demonstrate the representation and classification ability of the comb-based PNP (see Appendix C). To investigate the comb power distributions of similar input frequencies, the actual input frequencies consist of accurate frequencies and a random variation value.
B. Experimental Demonstration
The experimental setup of the comb-based PNP is presented in Fig. 2. A schematic of the PNP is shown on the upper side. The node colors of different layers are in accordance with the background color of experimental stages on the lower side. The PNP is generated by an electro-optical dual-OFC scheme, where the output of a narrow-linewidth continuous-wave laser (CW, TLG-200, Alnair Labs) is split into two branches to be respectively modulated through phase modulators (PMs, PM-0K5-10-PFA-PFA, EOspace). Then the central wavelength of one OFC is shifted for 100 MHz by an acousto-optical modulator (AOM, SGTF100-1550-1, Smart Sci and Tech). Using two phase-locked RF channels of a signal generator (N5183B, Keysight), the modulation frequencies denoted as
Figure 2.Experimental setup and corresponding schematic of the comb-based PNP. On the upper side, the input layer connects to the PNP layer by carrier coupling. The gain competition inside the PNP resembles the lateral inhibition connection in the biological counterpart [27]. The output layer represents the beat notes for the PNP activities monitoring. On the lower side, an electro-optical dual-OFC with slightly different comb spacings is generated by parallel PMs. The frequency-shifted OFC is optically injected into a DFB-LD and the PC is used for injection efficiency optimization. The output of the DFB-LD is monitored by an OSA and then detected in a low-speed PD with the other OFC. CW, continuous-wave laser; PM, phase modulator; AOM, acousto-optical modulator; PC, polarization controller; DFB-LD, distributed-feedback laser diode; OSA, optical spectrum analyzer; VOA, variable optical attenuator; PD, photodetector; ESA, electrical spectrum analyzer.
The frequency-shifted OFC is injected into a DFB-LD (T-20-550-B-07#G, Henan Shijia) with no optical isolator at the output port through an optical circulator and a polarization controller (PC). A DFB-LD with a modulation bandwidth of 20 GHz is provided for input RF signals. As a result, the carrier coupling provides the inter-layer connection (i.e., layer i and layer ii) and the intra-layer connection (i.e., inside layer i). It is known that both the optical injection and direct modulation can introduce a redshift of the central wavelength of the DFB-LD, which is dependent on the injection power or modulation depth [29]. Hence, a relatively low input power (SMA-100B, Rohde and Schwarz) can reduce the effect of the unwanted detuning wavelength. Another advantage is the elimination of modulation harmonics, which may disturb the distributed response of the comb neuron. The output of the DFB-LD is divided into two parts for monitoring by an optical spectrum analyzer (OSA, AQ6370C, Yokogawa) and coupling with the other OFC for heterodyne interference, respectively. The electric signal of a 500 MHz PD (FS-PD-B-2030, Fsphotonics) laid after the heterodyne interference is monitored by an electrical spectrum analyzer (ESA, Rohde and Schwarz) centered at 100 MHz (i.e., the shifted frequency by the AOM) with a 500 Hz resolution bandwidth for the readout of the comb power variation through the beat notes at
Given that the CW laser and DFB-LD operate independently with noise and temperature drift, it is critical to keep their detuning frequency (i.e.,
3. RESULTS
A. Photonic Tuning Curve of Comb Neurons
The responses of a comb neuron to a single input frequency are given in Fig. 3. The CW laser is centered at the red-side of the DFB-LD with a wavelength difference of 0.1 nm and an optical output of 15 dBm. We apply a 25 dBm RF power for dual-OFC generation with six beat notes as the comb neuron signatures. The
Figure 3.Schematics and corresponding experimental results of the comb neuron response for a single input frequency. (a)–(d) The illustrative cases of no input, 6.6 GHz input, 9.6 GHz input, and 12.4 GHz input, respectively. The input frequency falls into response ranges of respective comb neurons and leads to a variation of the comb power. (e)–(h) Optical spectra of different input cases. The dotted box in red highlights the apparent decrease of corresponding comb power. (i)–(l) Electrical spectra of different input cases. The comb powers are monitored through the beat note powers. The center frequency and the spacing of the beat notes correspond to the frequency shift and frequency difference of the OFCs, respectively.
Figures 3(a)–3(d) present the schematics of response cases corresponding to various input frequency bands. We focus on the central comb neuron and the adjacent two comb neurons, denoted as comb 0, comb
We scan the input frequency to investigate the response curve of comb neurons. In Fig. 4, the beat note powers corresponding to different input frequencies are given. First, we find that each comb neuron particularly responds to the input frequency within an adjacent range. It indicates that the frequency information of the input can be inferred from the variation of the comb power. This nonlinear response resembles an inverse bell-shape tuning curve like in biological neurons. Second, the width and amplitude (i.e., the maximum variation of comb power) of the response curve are dependent on the initial comb power. A relatively flat response curve of comb 0 shows a larger response width and a lower response amplitude compared to those of comb 1 and comb
Figure 4.Beat note powers dependent on input frequencies. The response range locates around the multiple times of the comb spacing. The inverse bell-shape response curve of each comb neuron resembles the tuning curve of biological neurons. The response width and amplitude are related to the initial comb power.
Time stability of the comb neuron response is studied by comparing the beat note powers under identical input after a period of time (see Appendix D). The stable response manifests the feasibility of the pre-calibration method for eliminating the effect of drifting
Further investigation on the shapes of response curves is presented and discussed in Appendix B. The inverse bell-shape tuning curves are numerically demonstrated, which agree well with the experimental results in Fig. 4. In addition, other shapes of response curves with both positive and negative peaks are also theoretically and experimentally observed. The comb power starts to rise as the input frequency scans, then falls below the initial level, and gradually recovers to the initial level. It indicates the comb neurons can provide both excitatory and inhibitory responses to an input frequency. Moreover, it is found that the response width and amplitude are also dependent on the input RF power.
B. Comb Power Coding for Input Pattern Classification
A classification task of three input patterns with dual RF tones is implemented with the comb-based PNP. An arbitrary waveform generator (M8195A, Keysight) outputs a single RF tone with an amplitude of 600 mV, which is subsequently filtered by a bandpass filter (BPF, BPF6-10Gs-2976, RF Bay) to remove the spurs. Another 4 dBm RF tone is produced by the RF signal generator and combined with the output of BPF as the input of the DFB-LD.
We prepare the inputs through a combination of random tones around the three frequency bands of 6 GHz, 9 GHz, and 12 GHz corresponding to the response ranges of the comb neurons. The experimental results of the relative beat note powers are shown in Fig. 5. The faded circles represent the PNP activities for each input. The stars indicate the average activities for three input patterns that are in red, green, and purple. Additionally, the projections of the average activities to the planes are marked. It is found that every input pattern occupies adjacent positions in this high-dimensional state space created by the comb powers. It manifests that the comb-based PNP has approximate responses to similar inputs. For example, when band 1 and band 2 are involved in the input, beat power 1, beat power 2, and beat power 3 decrease, rise, and remain invariant, respectively. Consequently, the comb powers function as a set of state variables mapping onto the input pattern and the PNP activities form three distinct clusters, which can be read out for classification through simple linear regression in a time-efficient manner. Here, this process is performed offline to derive the classification results in the electrical domain. Note that the nonlinear response supports the distributed representation of the information in each RF tone by the comb-based PNP. As a result, an input RF pattern could reliably map onto the distribution of the comb power. This process can be considered as a feature conversion, which benefits from the readability of the comb power by a low-speed PD. Therefore, we can efficiently classify the input RF patterns.
Figure 5.Comb-based PNP activities of three input patterns with dual radio-frequency (RF) tones. The tones are randomly selected from three frequency bands. The PNP shows similar activities in response to the input pattern with approximate frequencies. Classification results can be consequently read out.
As the number of comb neurons and input frequencies increases, the response of the PNP is nontrivial considering the intra-cavity interaction and effects such as gain competition, which may lead to more complex and unique PNP activities. In the numerical investigations, the PNP activities of 15 comb neurons for four input patterns with 15 RF tones are compared (see Appendix C). The results show that a comb power increases (i.e., an excitatory response) if there is an RF tone around itself, which means the comb-based PNP can process the input pattern in a parallel and distributed style. Therefore, the input patterns are successfully classified according to the PNP activities.
4. CONCLUSION
We have demonstrated a comb-based PNP to achieve a population-based paradigm for parallel and nonlinear processing. The implementations of population generation, parallel processing, and population activity reading out are introduced. Furthermore, we have presented the distributed response of comb neurons as photonic tuning curves, and comb power coding for input pattern classification. Three features are highlighted for the comb-based PNP. First, it keeps a low physical complexity toward a large population with the PNP-tunability and activity-readability. The promising OFC technology ensures a chance of the comb-based PNP to scale up with abundant bandwidth resources [23]. The PNP can also be efficiently tuned and read out instead of accessing to individual neurons one by one. Second, the carrier-coupling naturally creates the complex interconnection with compactness. A similarity between gain competition and lateral inhibition is interesting for potential higher-level population-based processing [30]. Third, it enables an RF pattern processing directly in the frequency domain owing to the neuron-like nonlinear response, which is distinct from other reported comb-based processing paradigms. For example, the comb-based optical neural network [31,32] focuses on the temporal processing of the data waveform with linear operations, such as the synaptic weighting and the linear mapping between the wavelength and delay. Similarly, the comb-based RF processing also relies on the linear manipulation of the amplitude and the relative delay of the comb lines for typical signal processing functions like RF filters [33]. To take a step further, we consider the comb-based PNP in the context of the conventional photonics-assisted RF signals characterization [22]. Generally, a linear mapping is constructed between the RF domain and the spatial or temporal domain [34,35]. It introduces increased physical complexity or extra response latency (i.e., a scanning period). In applications such as spectrum monitoring and ultra-fast frequency-hopping communication, the response latency of RF signals characterization is critical for the performance.
The comb-based PNP relies on the nonlinear mapping of the broad input RF frequencies onto the distributed comb powers, which can be readily monitored and conveniently configured. Therefore, the comb-based PNP enables an improved efficiency in the pattern classification task in the RF domain compared to the conventional schemes. For example, when dealing with broadband and sparse RF signals, the conventional schemes are less efficient as most of the time resources and channels are wasted without obtaining valuable information. However, the comb-based PNP can directly sense the dynamical change of the RF signals with just a series of comb powers. In future studies, the PNP generation can be improved to generate more comb neurons and equipped with a programmable power distribution through a wave-shaper. The pre-calibration method can be implemented with a feedback control to efficiently guarantee the stability. It is also interesting to study the PNP interconnection dependent on cavity properties for a better understanding of the network topology. At last, recent breakthroughs of substantially efficient on-chip OFC [36,37] indicate the potential monolithic integration of the comb-based PNP.
APPENDIX A: THEORETICAL MODEL
The dynamics of an SL when it is injected by an OFC and simultaneously driven by an input RF signal is described. The dynamics characterization is based on the amplitude
APPENDIX B: CHARACTERIZATION OF PHOTONIC TUNING CURVE
To characterize the response of the comb power to a single input frequency (i.e., photonic tuning curve), we scan the
Figure 6.Characterization of shapes of the photonic tuning curve. (a)–(c) Numerical results of the inverse bell-shape, dual-peak shape, distributed dual-peak shape, respectively. (d)–(f) Experimental results of the distributed dual-peak shape, dual-peak shape, and input-power dependence.
APPENDIX C: CONFIGURATION OF COMPLEX PATTERNS CLASSIFICATION
To numerically investigate the response to complex input patterns, we consider a classification task of four input patterns of 15 input tones and record the optical spectrum of 15 combs separated by 150 MHz, as shown in Fig.
Figure 7.Numerical results of classification of the complex input patterns. (a) Schematic of the classification task of four input patterns with 15 tones. (b)–(e) Optical spectra corresponding to the four input patterns, respectively. The sequence numbers of the lower comb powers are indicated.
APPENDIX D: PRE-CALIBRATION METHOD
We implement a pre-calibration experimental method to overcome the drifting of
Figure 8.Experimental results of the pre-calibration method. (a), (b) The state comb power increases as the detuning frequency (
References
[1] W. Ma, J. M. Beck, P. E. Latham, A. Pouget. Bayesian inference with probabilistic population codes. Nat. Neurosci., 9, 1432-1438(2006).
[2] T. Womelsdorf, K. Anton-Erxleben, F. Pieper, S. Treue. Dynamic shifts of visual receptive fields in cortical area MT by spatial attention. Nat. Neurosci., 9, 1156-1160(2006).
[3] A. Pouget, P. Dayan, R. Zemel. Information processing with population codes. Nat. Rev. Neurosci., 1, 125-132(2000).
[4] K. Roth, S. Shao, J. Gjorgjieva. Efficient population coding depends on stimulus convergence and source of noise. PLoS Comput. Biol., 17, e1008897(2021).
[5] J. Grollier, D. Querlioz, K. Y. Camsari, K. Everschor-Sitte, S. Fukami, M. D. Stiles. Neuromorphic spintronics. Nat. Electron., 3, 360-370(2020).
[6] A. Mizrahi, T. Hirtzlin, A. Fukushima, H. Kubota, S. Yuasa, J. Grollier, D. Querlioz. Neural-like computing with populations of superparamagnetic basis functions. Nat. Commun., 9, 1533(2018).
[7] M. Romera, P. Talatchian, S. Tsunegi, F. A. Araujo, V. Cros, P. Bortolotti, J. Trastoy, K. Yakushiji, A. Fukushima, H. Kubota, S. Yuasa, M. Ernoult, D. Vodenicarevic, T. Hirtzlin, N. Locatelli, D. Querlioz, J. Grollier. Vowel recognition with four coupled spin-torque nano-oscillators. Nature, 563, 230-234(2018).
[8] G. Wetzstein, A. Ozcan, S. Gigan, S. Fan, D. Englund, M. Soljačić, C. Denz, D. A. B. Miller, D. Psaltis. Inference in artificial intelligence with deep optics and photonics. Nature, 588, 39-47(2020).
[9] B. Ma, W. Zou. Demonstration of a distributed feedback laser diode working as a graded-potential-signaling photonic neuron and its application to neuromorphic information processing. Sci. China Inf. Sci., 63, 160408(2020).
[10] M. A. Nahmias, B. J. Shastri, A. N. Tait, P. R. Prucnal. A leaky integrate-and-fire laser neuron for ultrafast cognitive computing. IEEE J. Sel. Top. Quantum Electron., 19, 1800212(2013).
[11] S. Xiang, Z. Ren, Z. Song, Y. Zhang, X. Guo, G. Han, Y. Hao. Computing primitive of fully VCSEL-based all-optical spiking neural network for supervised learning and pattern classification. IEEE Trans. Neural Netw. Learn. Syst., 32, 2494-2505(2020).
[12] G. Sarantoglou, M. Skontranis, C. Mesaritakis. All optical integrate and fire neuromorphic node based on single section quantum dot laser. IEEE J. Sel. Top. Quantum Electron., 26, 1900310(2020).
[13] V. A. Pammi, K. Alfaro-Bittner, M. G. Clerc, S. Barbay. Photonic computing with single and coupled spiking micropillar lasers. IEEE J. Sel. Top. Quantum Electron., 26, 1500307(2020).
[14] J. Robertson, E. Wade, Y. Kopp, J. Bueno, A. Hurtado. Toward neuromorphic photonic networks of ultrafast spiking laser neurons. IEEE J. Sel. Top. Quantum Electron., 26, 7700715(2020).
[15] A. N. Tait, T. F. de Lima, M. A. Nahmias, H. B. Miller, H. T. Peng, B. J. Shastri, P. R. Prucnal. Silicon photonic modulator neuron. Phys. Rev. Appl., 11, 064043(2019).
[16] J. Feldmann, N. Youngblood, C. D. Wright, H. Bhaskaran, W. H. P. Pernice. All-optical spiking neurosynaptic networks with self-learning capabilities. Nature, 569, 208-214(2019).
[17] T. Van Vaerenbergh, M. Fiers, P. Mechet, T. Spuesens, R. Kumar, G. Morthier, B. Schrauwen, J. Dambre, P. Bienstman. Cascadable excitability in microrings. Opt. Express, 20, 20292-20308(2012).
[18] G. Van der Sande, D. Brunner, M. C. Soriano. Advances in photonic reservoir computing. Nanophotonics, 6, 561-576(2017).
[19] T. Inagaki, K. Inaba, T. Leleu, T. Honjo, T. Ikuta, K. Enbutsu, T. Umeki, R. Kasahara, K. Aihara, H. Takesue. Collective and synchronous dynamics of photonic spiking neurons. Nat. Commun., 12, 2325(2021).
[20] B. J. Shastri, A. N. Tait, T. F. de Lima, W. H. P. Pernice, H. Bhaskaran, C. D. Wright, P. R. Prucnal. Photonics for artificial intelligence and neuromorphic computing. Nat. Photonics, 15, 102-114(2021).
[21] E. Khoram, A. Chen, D. Liu, L. Ying, Q. Wang, M. Yuan, Z. Yu. Nanophotonic media for artificial neural inference. Photon. Res., 7, 823-827(2019).
[22] L. R. Cortes, D. Onori, H. G. de Chatellus, M. Burla, J. Azana. Towards on-chip photonic-assisted radio-frequency spectral measurement and monitoring. Optica, 7, 434-447(2020).
[23] A. E. Willner, A. Fallahpour, K. Zou, F. Alishahi, H. Zhou. Optical signal processing aided by optical frequency combs. IEEE J. Sel. Top. Quantum Electron., 27, 7700916(2021).
[24] I. Coddington, N. Newbury, W. Swann. Dual-comb spectroscopy. Optica, 3, 414-426(2016).
[25] Y. Doumbia, T. Malica, D. Wolfersberger, K. Panajotov, M. Sciamanna. Optical injection dynamics of frequency combs. Opt. Lett., 45, 435-438(2020).
[26] Y. Doumbia, T. Malica, D. Wolfersberger, K. Panajotov, M. Sciamanna. Nonlinear dynamics of a laser diode with an injection of an optical frequency comb. Opt. Express, 28, 30379-30390(2020).
[27] A. C. Arevian, V. Kapoor, N. N. Urban. Activity-dependent gating of lateral inhibition in the mouse olfactory bulb. Nat. Neurosci., 11, 80-87(2008).
[28] H. Francis, X. Zhang, S. Chen, J. Yu, K. Che, M. Hopkinson, C. Jin. Optical frequency comb generation via cascaded intensity and phase photonic crystal modulators. IEEE J. Sel. Top. Quantum Electron., 27, 2100209(2021).
[29] A. Murakami, K. Kawashima, K. Atsuki. Cavity resonance shift and bandwidth enhancement in semiconductor lasers with strong light injection. IEEE J. Quantum Electron., 39, 1196-1204(2003).
[30] Y. Zhang, S. Xiang, X. Guo, A. Wen, Y. Hao. The winner-take-all mechanism for all-optical systems of pattern recognition and max-pooling operation. J. Lightwave Technol., 38, 5071-5077(2020).
[31] W. Zou, B. Ma, S. Xu, X. Zou, X. Wang. Towards an intelligent photonic system. Sci. China Inf. Sci., 63, 160401(2020).
[32] X. Xu, M. Tan, B. Corcoran, J. Wu, T. G. Nguyen, A. Boes, S. T. Chu, B. E. Little, R. Morandotti, A. Mitchell, D. G. Hicks, D. J. Moss. Photonic perceptron based on a Kerr microcomb for high-speed, scalable, optical neural networks. Laser Photonics Rev., 14, 2000070(2020).
[33] X. Xu, M. Tan, J. Wu, R. Morandotti, A. Mitchell, D. J. Moss. Microcomb-based photonic RF signal processing. IEEE Photonics Technol. Lett., 31, 1854-1857(2019).
[34] Y. Duan, L. Chen, H. Zhou, X. Zhou, C. Zhang, X. Zhang. Ultrafast electrical spectrum analyzer based on all-optical Fourier transform and temporal magnification. Opt. Express, 25, 7520-7529(2017).
[35] X. Xie, Y. Dai, Y. Ji, K. Xu, Y. Li, J. Wu, J. Lin. Broadband photonic radio-frequency channelization based on a 39-GHz optical frequency comb. IEEE Photonics Technol. Lett., 24, 661-663(2012).
[36] C. Xiang, J. Liu, J. Guo, L. Chang, R. Wang, W. Weng, J. Peters, W. Xie, Z. Zhang, J. Riemensberger, J. Selvidge, T. J. Kippenberg, J. E. Bowers. Laser soliton microcombs heterogeneously integrated on silicon. Science, 373, 99-103(2021).
[37] J. Liu, G. Huang, R. Wang, J. He, A. S. Raja, T. Liu, N. J. Engelsen, T. J. Kippenberg. High-yield, wafer-scale fabrication of ultralow-loss, dispersion-engineered silicon nitride photonic circuits. Nat. Commun., 12, 2236(2021).
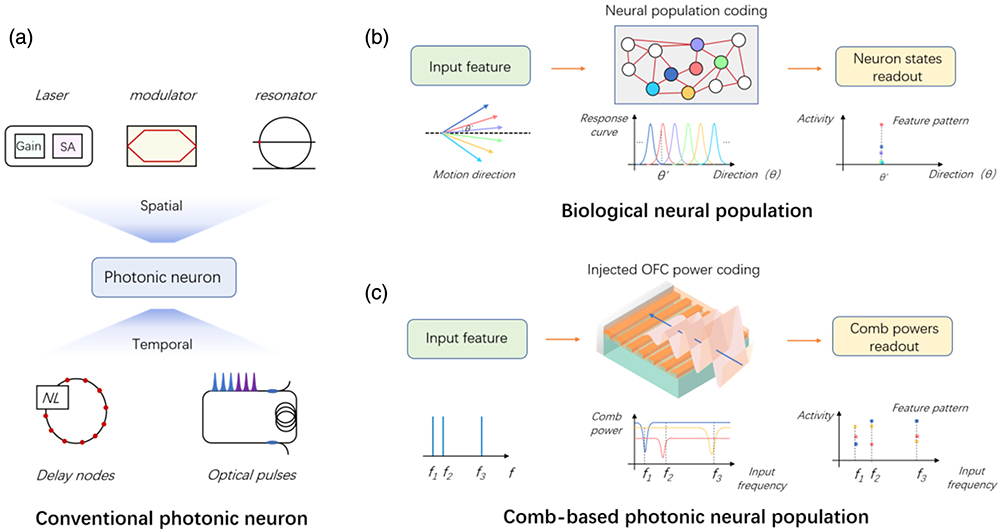
Set citation alerts for the article
Please enter your email address