SUN Hong, LIU Ning, WU Li, ZHENG Tao, LI Min-zan, WU Jing-zhu. Visualization of Water Content Distribution in Potato Leaves Based on Hyperspectral Image[J]. Spectroscopy and Spectral Analysis, 2019, 39(3): 910

Search by keywords or author
- Spectroscopy and Spectral Analysis
- Vol. 39, Issue 3, 910 (2019)
Abstract
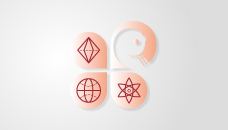
Set citation alerts for the article
Please enter your email address