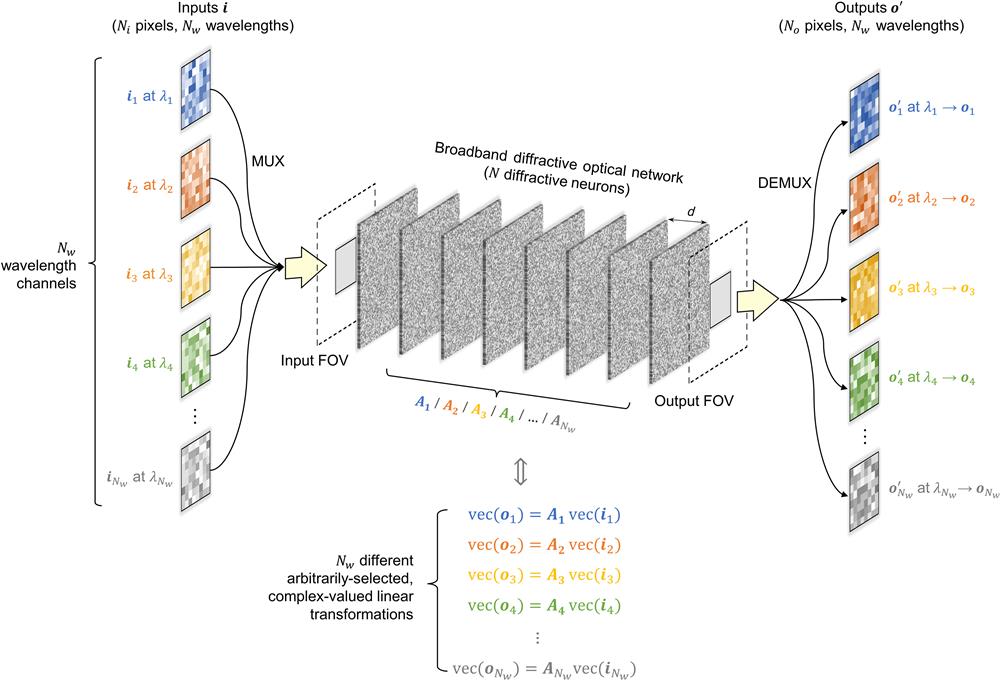
Fig. 1. Schematic of massively parallel, wavelength-multiplexed diffractive optical computing. Optical layout of the wavelength-multiplexed diffractive neural network, where the diffractive layers are jointly trained to perform different arbitrarily selected, complex-valued linear transformations between the input field and the output field using wavelength multiplexing. The optical fields at the input FOV, , are encoded at a predetermined set of distinct wavelengths , respectively, using a wavelength multiplexing (“MUX”) scheme. At the output FOV of the broadband diffractive network, wavelength demultiplexing (“DEMUX”) is performed to extract the diffractive output fields at the corresponding wavelengths , respectively, which represent the all-optical estimates of the target output fields , corresponding to the target linear transformations (). Through this diffractive architecture, different arbitrarily selected complex-valued linear transformations can be all-optically performed at distinct wavelengths, running in parallel channels of the broadband diffractive processor.
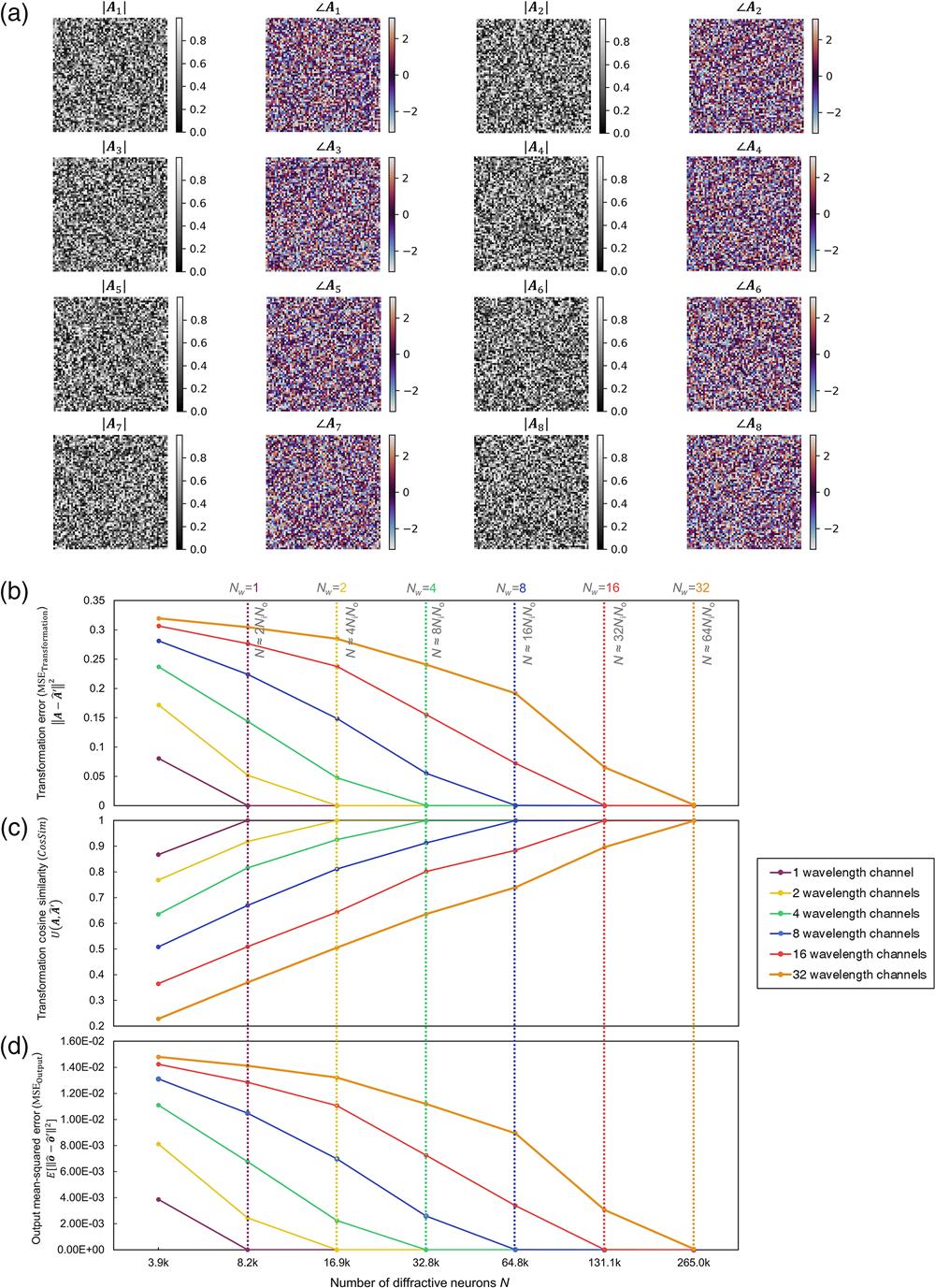
Fig. 2. All-optical transformation performances of broadband diffractive networks using different numbers of wavelength channels. (a) As examples, we show the amplitude and phase of the first eight matrices in that were randomly generated, serving as the ground truth (target) for the diffractive all-optical transformations. See Fig. S1 in the Supplementary Material for the cosine similarity values calculated between any two combinations of these 32 target linear transformation matrices. (b) The mean values of the normalized MSE between the ground-truth transformation matrices () and the corresponding all-optical transforms () across different wavelength channels are reported as a function of the number of diffractive neurons . The results of the diffractive networks using different numbers of wavelength channels are encoded with different colors, and the space between the simulation data points is linearly interpolated. ∈ {1, 2, 4, 8, 16, and 32}, ∈ {3.9k, 8.2k, 16.9k, 32.8k, 64.8k, 131.1k, 265.0k} and . (c) Same as (b) but the cosine similarity values between the all-optical transforms and their ground truth are reported. (d) Same as (b) but the MSE values between the diffractive network output fields and the ground-truth output fields are reported.
Fig. 3. All-optical transformation performances of the individual wavelength channels in broadband diffractive network designs with and . The output field errors for the all-optical linear transforms achieved by the wavelength-multiplexed diffractive network models with (a) 2-channel wavelength multiplexing (), ; (b) 4-channel wavelength multiplexing (), ; (c) 8-channel wavelength multiplexing (), ; (d) 16-channel wavelength multiplexing (), ; and (e) 32-channel wavelength multiplexing (), . The standard deviations (error bars) of these metrics are calculated across the entire testing data set.
Fig. 4. All-optical transformation matrices estimated by two different wavelength-multiplexed broadband diffractive networks with and . The first broadband diffractive network has trainable diffractive neurons. The second broadband diffractive network has trainable diffractive neurons. The absolute differences between these all-optical transformation matrices and the corresponding ground-truth (target) matrices are also shown in each case. diffractive design achieves a much smaller and negligible absolute error due to the increased degrees of freedom.
Fig. 5. Examples of the input/output complex fields for the ground-truth (target) transformations along with the all-optical output fields resulting from the 8-channel wavelength-multiplexed diffractive design using . Absolute errors between the ground-truth output fields and the all-optical diffractive network output fields are negligible. Note that indicates the wrapped phase difference between the ground-truth output field and the normalized diffractive network output field .
Fig. 6. Exploration of the limits of the number of wavelength channels that can be implemented in a broadband diffractive network. (a) The mean values of the normalized MSE between the ground-truth transformation matrices () and the all-optical transforms () across different wavelength channels are reported as a function of . The results of the broadband diffractive networks using different numbers of diffractive neurons () are presented with different colors: . Dotted lines are fitted based on the data points whose diffractive networks share the same . (b) Same as (a) but the cosine similarity values between the all-optical transforms and their ground truth are reported. (c) Same as (a) but the MSE values between the diffractive network output fields and the ground-truth output fields are reported. .
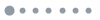
Fig. 7. The impact of material dispersion and losses on the all-optical transformation performance of wavelength-multiplexed broadband diffractive networks. (a) The mean values of the normalized MSE between the ground-truth transformation matrices () and the all-optical transforms () across different wavelength channels are reported as a function of the material of the diffractive layers. The results of the diffractive networks trained with and without diffraction efficiency penalty are presented in yellow and purple colors, respectively. , , and . (b) Same as (a) but the cosine similarity values between the all-optical transforms and their ground truth are reported. (c) Same as (a) but the MSE values between the diffractive network output fields and the ground-truth fields are reported. (d) The mean diffraction efficiencies of the presented diffractive models across all the wavelength channels. (e) Diffraction efficiency of the individual wavelength channels for the broadband diffractive network model presented in (a)–(d) that uses the dielectric material without the diffraction efficiency-related penalty term in its loss function. (f) Same as (e), but the diffractive network was trained using a loss function with the diffraction efficiency-related penalty term.
Fig. 8. All-optical transformation performance of broadband diffractive network designs with , reported as a function of and the bit depth of the diffractive neurons. (a) The mean values of normalized MSE between the ground-truth transformation matrices () and the all-optical transforms () across different wavelength channels are reported as a function of . The results of the diffractive networks using different bit depths of the diffractive neurons, including 4, 8, 12, and 32, are encoded with different colors, and the space between the data points is linearly interpolated. , and . (b) Same as (a) but the cosine similarity values between the all-optical transforms and their ground truth are reported. (c) Same as (a) but the MSE values between the diffractive network output fields and the ground-truth output fields are reported.
Fig. 9. The impact of the encoding wavelength error on the all-optical linear transformation performance of a wavelength-multiplexed broadband diffractive network; , , and . (a) The normalized MSE values between the ground-truth transformation matrices () and the all-optical transforms () for the four different wavelength channels are reported as a function of the wavelengths used during the testing. The results of the different wavelength channels are shown with different colors, and the space between the simulation data points is linearly interpolated. (b) Same as (a) but the cosine similarity values between the all-optical transforms and their ground truth are reported. (c) Same as (a) but the MSE values between the diffractive network output fields and the ground-truth output fields are reported. The shaded areas indicate the standard deviation values calculated based on all the samples in the testing data set.
Fig. 10. An example of a wavelength-multiplexed diffractive network (, ) that all-optically performs eight different permutation (encoding) operations between its input and output FOVs, with each target permutation matrix assigned to a unique wavelength. (a) Input/output examples. Each one of the wavelength channels in the diffractive processor is assigned to a different permutation matrix . The absolute differences between the diffractive network output fields and the ground-truth (target) permuted (encoded) output fields are also shown in the last column. (b) Arbitrarily generated permutation matrices that serve as the ground truth (target) for the wavelength-multiplexed diffractive permutation transformations shown in (a).
Fig. 11. Experimental validation of a wavelength-multiplexed diffractive network with and . (a) Photograph of the experimental setup, including the schematic of the THz setup. (b) The fabricated wavelength-multiplexed diffractive processor. (c) The learned thickness profiles of the diffractive layers. (d) Photographs of the 3D-printed diffractive layers. (e) Experimental results of the diffractive processor for the two wavelength channels and using the fabricated diffractive layers, which reveal a good agreement with their numerical counterparts and the ground truth. .