Author Affiliations
1Institute of Quantum Technologies (IQT), The Hong Kong Polytechnic University, Hong Kong, China2Quantum Science and Engineering Centre (QSec), Nanyang Technological University, Singapore, Singapore3Departament de Fisica Quantica i Astrofisica and Institut de Ciencies del Cosmos (ICCUB), Universitat de Barcelona, Barcelona, Spain4Quantum Research Centre, Technology Innovation Institute, Abu Dhabi, UAE5Centre for Quantum Technologies, National University of Singapore, Singapore, Singapore6Institute of Microelectronics, A*STAR (Agency for Science, Technology and Research), Singapore, Singapore7Advanced Micro Foundry, Singapore, Singapore8National Institute of Education, Nanyang Technological University, Singapore, Singaporeshow less
Fig. 1. Schematic of the unary approach to option pricing, compared to the classical Monte Carlo method. (a) Integrated photonic chip with the unary algorithm, consisting of a generator of the generative adversarial network (GAN), payoff calculation, and quantum amplitude estimation for acceleration. (b) Monte Carlo simulation on a classical computer, which first generates the future asset price paths based on random variables, and then calculates the payoff. The accuracy relies on extensive simulations of random walk asset paths. (c) Expected acceleration of the convergence of payoff errors, compared to classical Monte Carlo simulations. Shaded areas in the top inset indicate statistical uncertainty.
Fig. 2. Mapping of asset prices to unary basis. (a) Classical Monte Carlo paths partitioned into different unary bases. (b) Probability density function (PDF) according to the defined unary basis. (c) Payoff value calculated according to the PDF and asset prices.
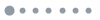
Fig. 3. Photonic chip design for the unary option pricing algorithm. (a) Algorithmic model of unary option pricing. The input state consists of an n-dimensional qudit and a two-dimensional ancilla. The following modules are contained: D, distribution loading; P, payoff calculation; Q, quantum operator for amplitude estimation. The amplification module Q is performed sequentially by Sψ→P†→D†→S0→D→P. The expected payoff is obtained by measuring the ancilla. (b) Optical circuit model by transforming the algorithmic model to linear optical operators. Each element of the unary basis is represented by two waveguides, extending the n-bin unary basis to 2n-dimensional Hilbert space. Relevant linear optical operators swp, Ry(θ), and XZX are listed with their waveguide structures. (c) Photonic chip design and architecture. The chip is designed by transforming the optical path model into waveguide structures and realizes the distribution loading, payoff calculation, and amplitude estimation sequentially. The distribution loading is trained as a GAN embedded in the machine learning module.
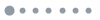
Fig. 4. GAN on the photonic chip for precise asset distribution uploading. (a) Algorithm of GAN, composed of a generator and a discriminator. (b) Generator implemented by a variational photonic circuit, which is trained on-chip in real time. The probability distributions accumulated on the waveguide paths are used as fake samples. Real samples are the training targets taken from market data in real applications. (c) Classical discriminator consisting of sequential convolutional layers and trained by a gradient descent algorithm. The discriminator aims to distinguish the source of the input sample, from the generator or a real distribution. The cost function is calculated from the discriminator output and used to train the discriminator itself and the generator. (d) The generator is trained by an evolutionary optimization procedure where populations (e.g., different configurations of the generator ansatz) are generated, evaluated, and iterated. The evaluation is accomplished using the scores granted by the discriminator. New generations are produced via the operators of selection, crossover, and mutation of current populations.
Fig. 5. Experimental training performance of the GAN under Wasserstein distance. (a), (c) Comparison between the probability distributions obtained experimentally from the generator (solid line with data points) and the target distribution (histogram). (b), (d) Evolution of the ℓ2 norm between the fake and real samples with increasing training iterations. (a), (b) Log-normal distribution; (c), (d) normal distribution.
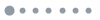
Fig. 6. Experimental results of option pricing with three asset values. (a) Illustration of the optical chip with payoff calculation and amplitude estimation module. Operator Q is repeated up to m (m≤50) times. The payoff is measured on waveguides that encode the ancilla in state |1⟩ when the asset price is larger than the pre-defined strike value. (b) Comparison between theoretical expectations and experimental results of the payoff, represented in angles. The raw angles (2m+1)θ are shifted back to the original angles θ, and the differences from theoretical expectations are recorded as errors. (c) Standard deviation (STD) of the expected payoff with increasing iterations of the amplitude estimation module. The STD converges from the initial ∼0.2 to less than 0.004. Iterations from 20 to 50 are zoomed in. (d) Error in payoff estimation between theoretical and experimental results, with increasing iterations of amplitude estimation. It shows a speedup in convergence compared to the Monte Carlo method.
Fig. 7. Fabricated quantum photonic chip.
Fig. 8. Simulation of the scaling of quantum AE and classical MC.