Liu Lixin, He Di, Li Mengzhu, Liu Xing, Qu Junle. Identification of Xinjiang Jujube Varieties Based on Hyperspectral Technique and Machine Learning[J]. Chinese Journal of Lasers, 2020, 47(11): 1111002

Search by keywords or author
- Chinese Journal of Lasers
- Vol. 47, Issue 11, 1111002 (2020)
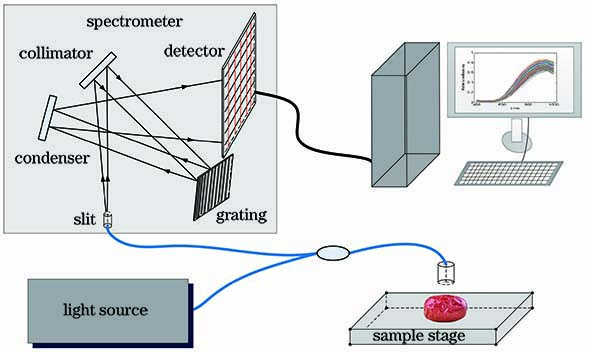
Fig. 1. Schematic of hyperspectral system
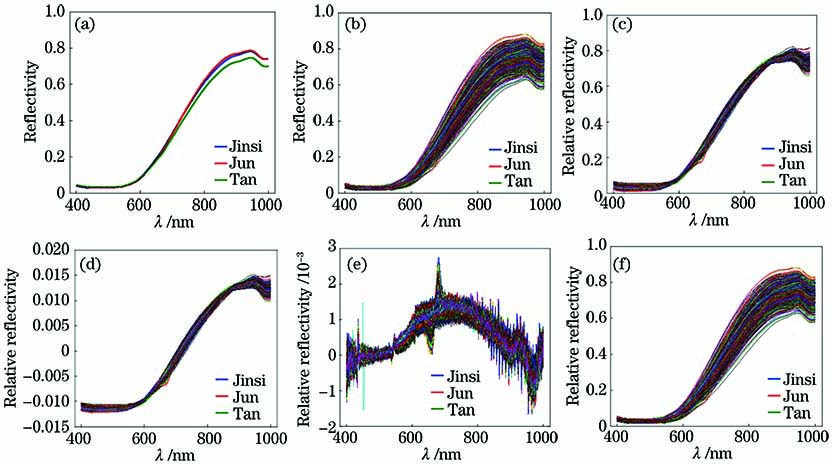
Fig. 2. Spectral profiles of Jujube samples before and after preprocess. (a) Average spectra; (b) original spectra; (c) preprocessed spectra by MSC; (d) preprocessed spectra by SNV; (e) preprocessed spectra by 1-Der; (f) preprocessed spectra by SG smoothing
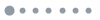
Fig. 3. Extracting characteristic bands by PCA. (a) Scores of the first three principal components; (b) variance contribution rate of the first ten principal components
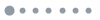
Fig. 4. Root-mean-square error calculated according to the number of selected characteristic variables
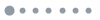
Fig. 5. Extracting characteristic bands by CARS. (a) Variation curve of the number of variables with the number of sampling; (b) variation curve of RMSECV with the number of sampling; (c) variation path of variable regression coefficient
|
Table 1. Identification results of LDA model with different pretreatment methods
|
Table 2. Identification results of KNN model with different pretreatment methods
|
Table 3. Identification results of SVM model with different pretreatment methods
|
Table 4. Identification results of LDA model with different characteristic bands extraction methods
|
Table 5. Identification results of KNN model with different characteristic bands extraction methods
|
Table 6. Identification results of SVM model with different characteristic bands extraction methods
|
Table 7. Accuracy and runtime of SVM model based on different characteristic bands extraction methods
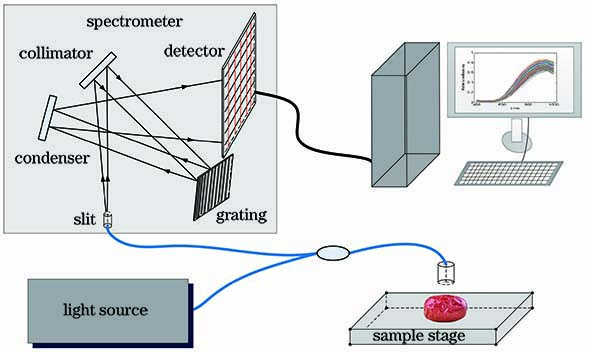
Set citation alerts for the article
Please enter your email address