Liu Lixin, He Di, Li Mengzhu, Liu Xing, Qu Junle. Identification of Xinjiang Jujube Varieties Based on Hyperspectral Technique and Machine Learning[J]. Chinese Journal of Lasers, 2020, 47(11): 1111002

Search by keywords or author
- Chinese Journal of Lasers
- Vol. 47, Issue 11, 1111002 (2020)
Abstract
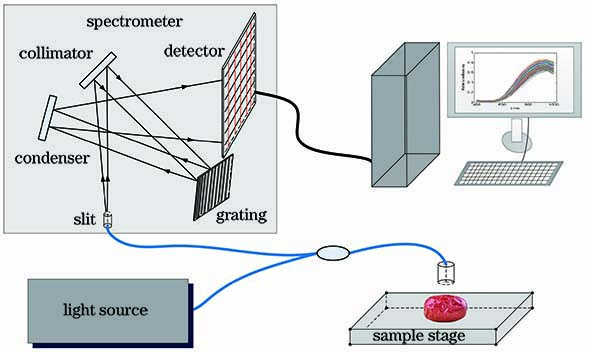
Set citation alerts for the article
Please enter your email address