Zhong-bao LIU, Jie WANG. Research on the Improvement of Spectra Classification Performance With the High-Performance Hybrid Deep Learning Network[J]. Spectroscopy and Spectral Analysis, 2022, 42(3): 699

Search by keywords or author
- Spectroscopy and Spectral Analysis
- Vol. 42, Issue 3, 699 (2022)
Abstract
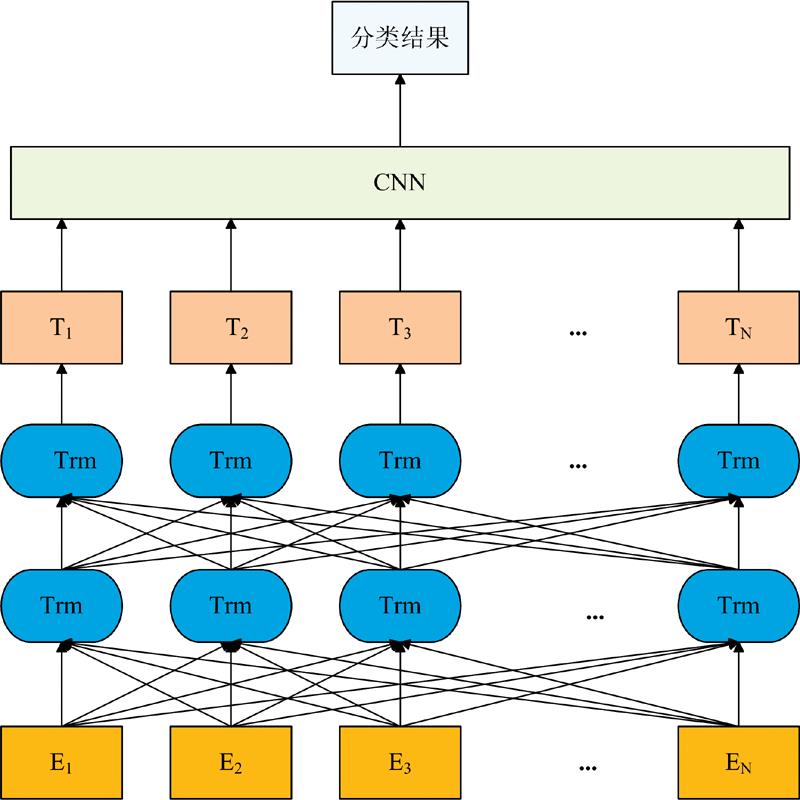
Set citation alerts for the article
Please enter your email address