HUANG Ying-lai, MENG Shi-yu, ZHAO Peng, YUE Meng-qiao. A Method for Identifying Wood Grades of Chinese Zither Panel Based on Near Infrared Spectroscopy[J]. Spectroscopy and Spectral Analysis, 2019, 39(3): 723

Search by keywords or author
- Spectroscopy and Spectral Analysis
- Vol. 39, Issue 3, 723 (2019)
Abstract
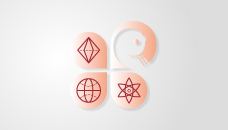
Set citation alerts for the article
Please enter your email address